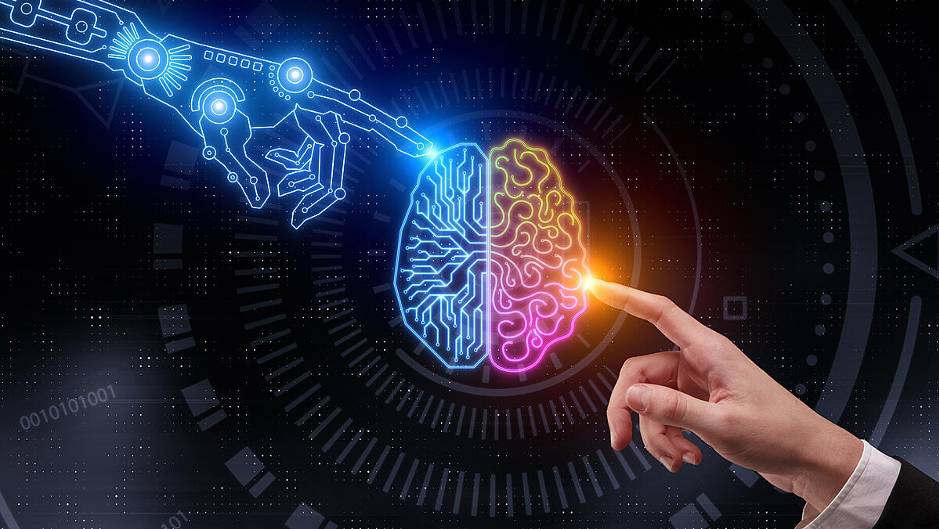
Beyond One-Size-Fits-All: Why Purpose-Built AI Elevates Customer Experiences to New Heights
In the age of digital transformation, artificial intelligence (AI) has become a cornerstone technology, driving innovations across various industries.
Among the plethora of applications, purpose-built AI stands out as particularly transformative in enhancing customer experiences. Unlike general AI that addresses broader needs, purpose-built AI is tailored for specific tasks or challenges within a business.
This specialization in functionality not only increases efficiency but also significantly improves the quality of customer interactions and satisfaction.
i. The Limitations of Generic AI
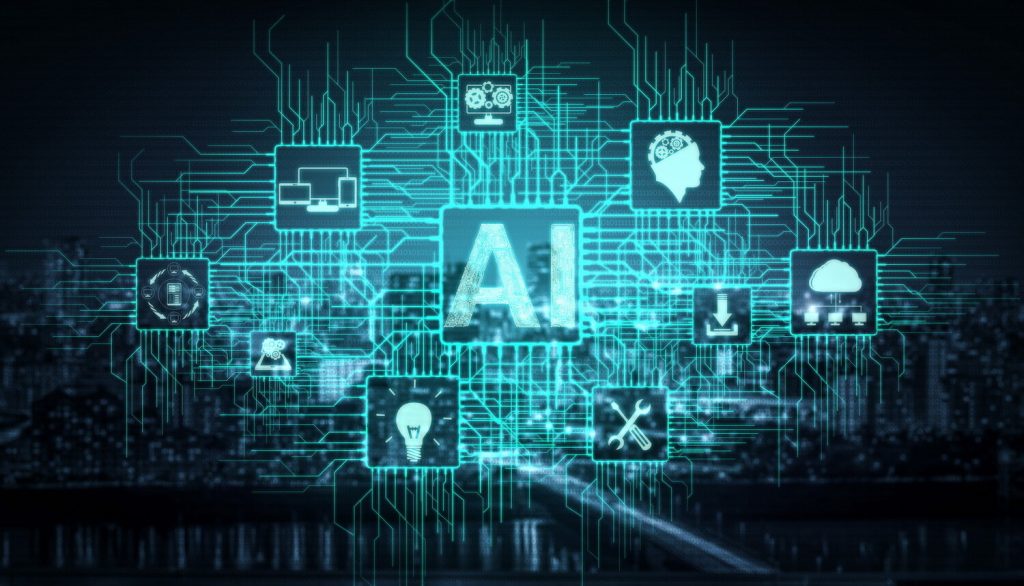
Traditional AI models are often trained on vast amounts of generic data. While these models can perform some customer service tasks, they may struggle to understand the nuances of specific industries or customer needs. This can lead to:
o Generic and impersonal interactions: Customers crave personalized experiences that cater to their unique needs and preferences. Generic AI can feel robotic and fail to connect on a deeper level.
o Inefficient problem-solving: Without a deep understanding of a specific domain, AI might struggle to identify and resolve complex customer issues effectively.
o Missed opportunities for personalization: Generic AI might miss opportunities to tailor recommendations, offers,or support based on individual customer behavior and preferences.
ii. What is Purpose-Built AI?
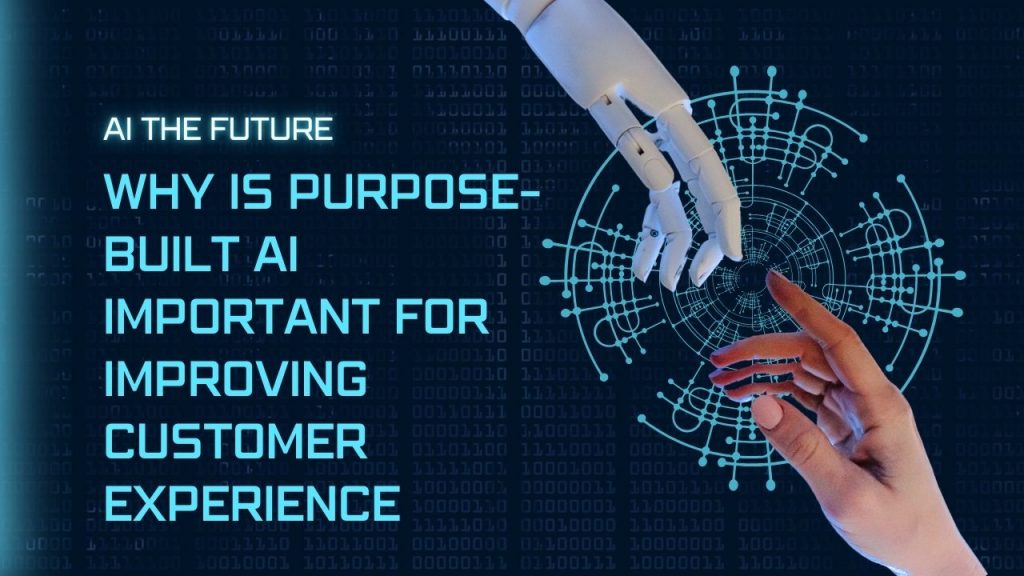
Purpose-built AI refers to systems that are designed and developed to solve a specific set of problems or to optimize certain processes. Unlike general AI, which aims at performing any cognitive task, purpose-built AI is highly specialized. Its architecture, data models, and algorithms are meticulously engineered to handle distinct tasks—from language processing in chatbots to predictive analytics in sales tools.
iii. The Power of Purpose-Built AI
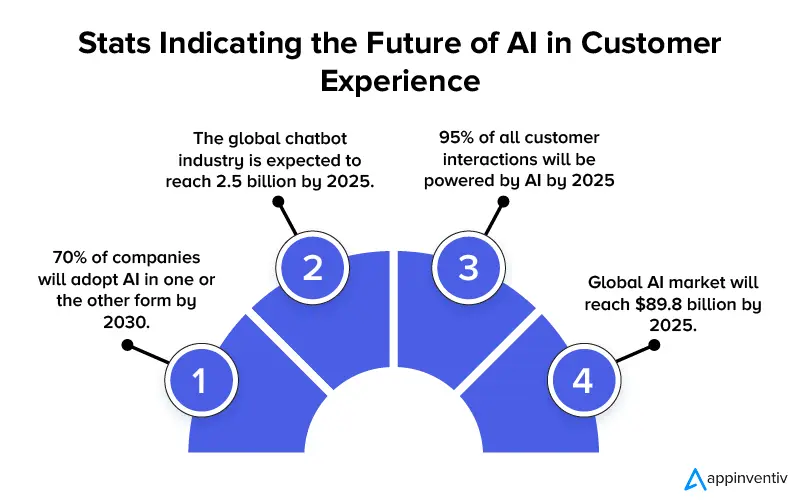
Purpose-built AI, on the other hand, is specifically designed for a particular industry or task. Here’s how it elevates the customer experience game:
o Deeper Domain Expertise: Trained on industry-specific data, purpose-built AI understands the unique language,challenges, and opportunities within a particular domain. This translates to more relevant interactions and problem-solving capabilities.
o Hyper-Personalization: Purpose-built AI can analyze customer data to anticipate needs, personalize recommendations, and offer targeted support, leading to a more satisfying customer journey.
o Responsiveness: AI enhances customer service interactions through chatbots and virtual assistants. These AI systems are programmed to handle routine inquiries efficiently and escalate more complex issues to human representatives. This not only speeds up response times but also frees up human agents to focus on higher-value interactions, improving overall service quality.
o Consistency: With purpose-built AI, businesses can ensure a consistent customer experience. AI systems do not suffer from human error and can maintain the same level of service across various points of contact. This consistency builds trust and reliability, encouraging customer loyalty.
o Improved Efficiency: By automating routine tasks and streamlining workflows, purpose-built AI empowers customer service agents to focus on complex issues and foster deeper customer connections.
iv. Streamlining Customer Service
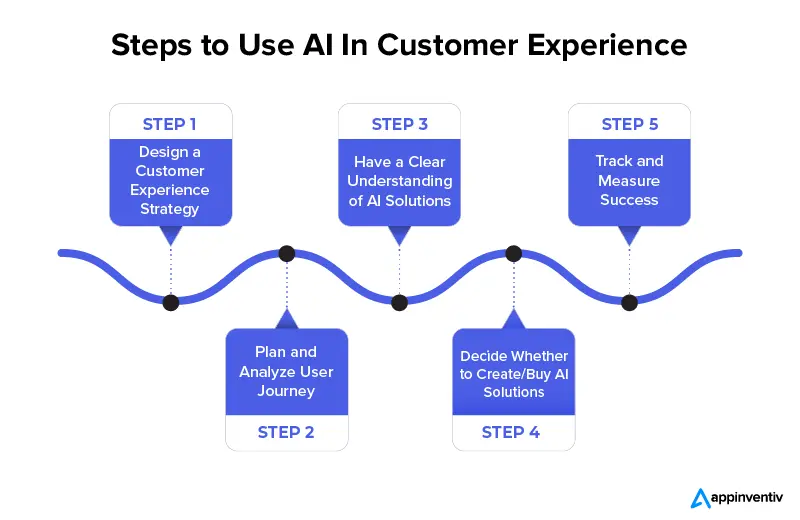
AI-powered chatbots and virtual assistants, designed specifically for customer service, can handle inquiries and issues efficiently, sometimes resolving scenarios without escalating them to human representatives. This rapid response leads to reduced wait times and higher customer satisfaction. Moreover, these systems can operate around the clock, providing constant support that significantly enhances overall customer service quality.
v. Predictive Analytics for Proactive Solutions
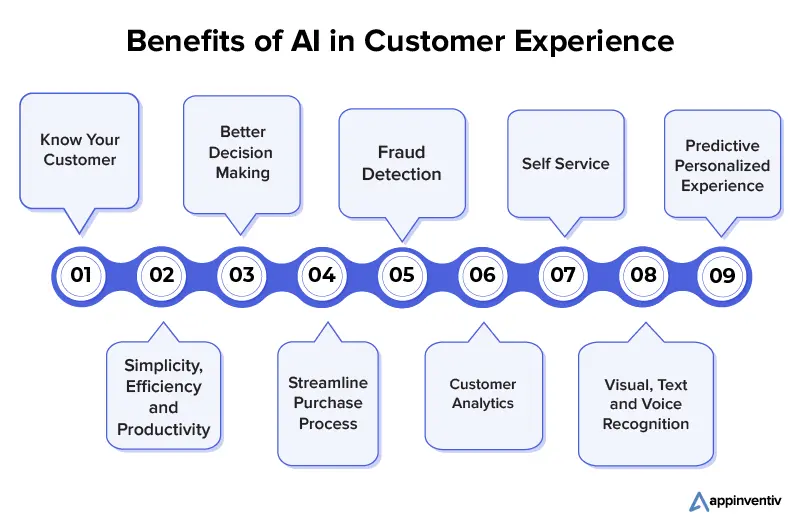
Purpose-built AI excels in predictive analytics, where AI systems analyze data to predict future trends and behaviors. This capability allows businesses to proactively address potential issues before they escalate or even anticipate customer needs. For example, if predictive analytics indicate that a customer may be experiencing issues with a product, proactive outreach can be initiated to offer support or a replacement, thus preventing dissatisfaction and building brand loyalty.
vi. Driving Operational Efficiency

By automating routine tasks, AI systems specifically developed for particular business functions can free up human workers to focus on more strategic, creative, or complex problems. This not only boosts productivity but also reduces human error and operational costs, ultimately impacting the business’s bottom line positively.
vii. Continuous Learning and Adaptation

Purpose-built AI systems are characterized by their ability to learn and adapt over time. They utilize machine learning algorithms to refine their operations based on new data, feedback, and outcomes. This continuous improvement cycle ensures that the customer experience is consistently becoming more effective and sophisticated.
viii. Implementation Examples in Industries
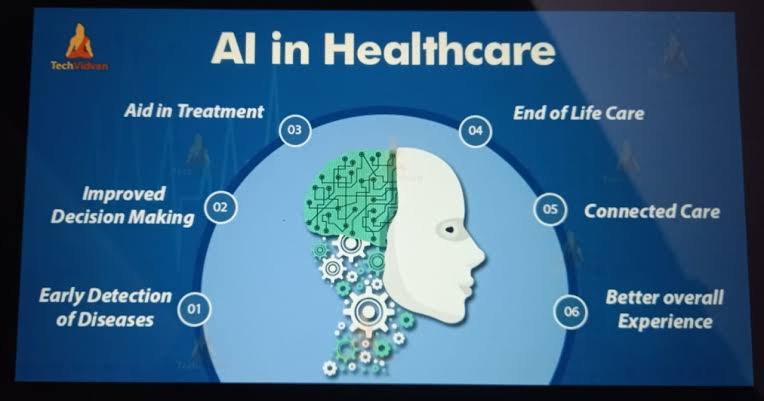
o Retail: Custom AI tools analyze consumer data to provide a curated shopping experience, manage inventories based on predictive analytics, and enhance customer service interactions through intelligent chatbots.
o Banking: AI systems designed for fraud detection not only protect customer assets but also increase their confidence in the security of their transactions. Additionally, AI-driven personalized financial advice adds significant value to customer interactions.
o Healthcare: AI applications in healthcare range from personalized patient care plans to AI-assisted diagnostics, significantly impacting patient satisfaction and outcomes.
o Travel and Hospitality: Tailored AI systems can manage bookings, provide personal travel recommendations, and predict peak demand periods for better resource allocation.
ix. Challenges and Considerations
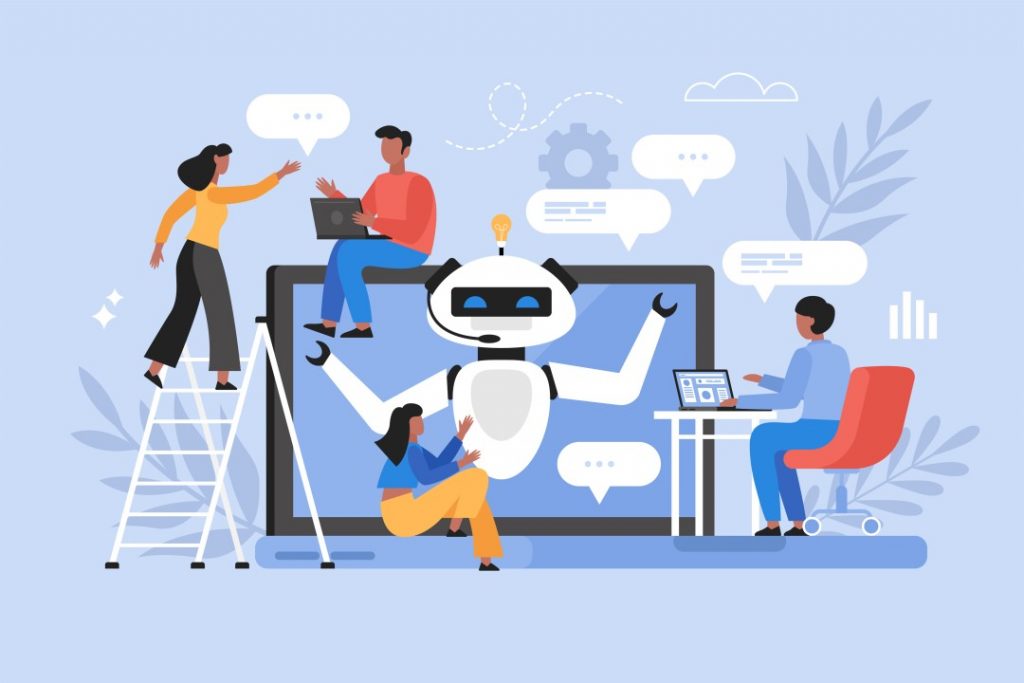
While the potential of purpose-built AI is immense, deploying these systems comes with its set of challenges.
Privacy concerns and ethical considerations must be carefully addressed to ensure that customer data is handled responsibly and transparently.
The need for constant updates, integration complexities, and ensuring AI ethics are adequately addressed are crucial considerations businesses must manage.
Moreover, the reliance on high-quality, extensive datasets for training these AI systems cannot be understated.
Without robust data, the effectiveness of purpose-built AI could be significantly limited, which emphasizes the importance of good data governance practices.
x. The Future of Customer Experience: A Symbiotic Relationship
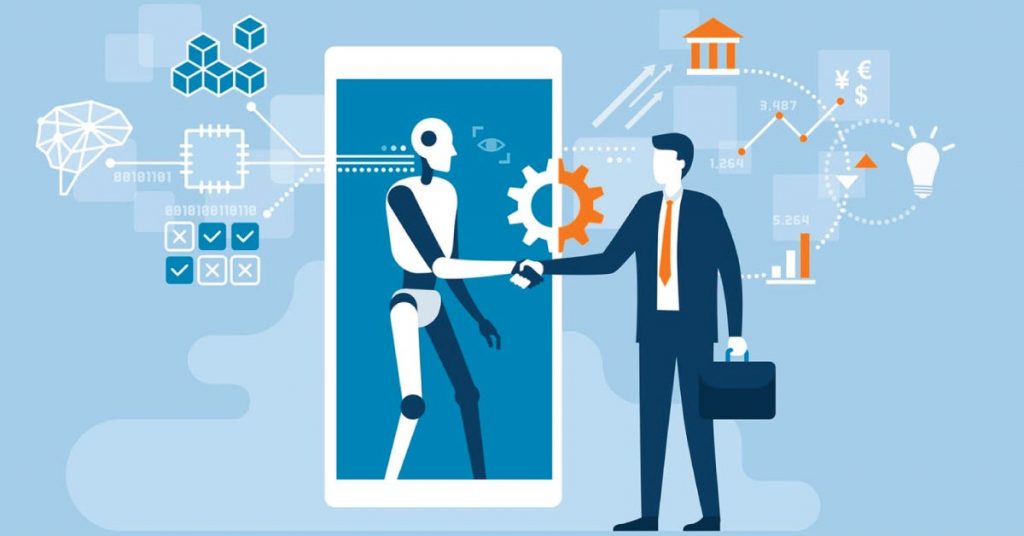
Purpose-built AI is not a replacement for human interaction; it’s a powerful tool to empower customer service teams. By leveraging AI’s deep domain knowledge and automation capabilities, human agents can focus on higher-level tasks like building rapport and resolving complex customer issues. This symbiotic relationship between human and machine paves the way for exceptional customer experiences.
xi. Conclusion
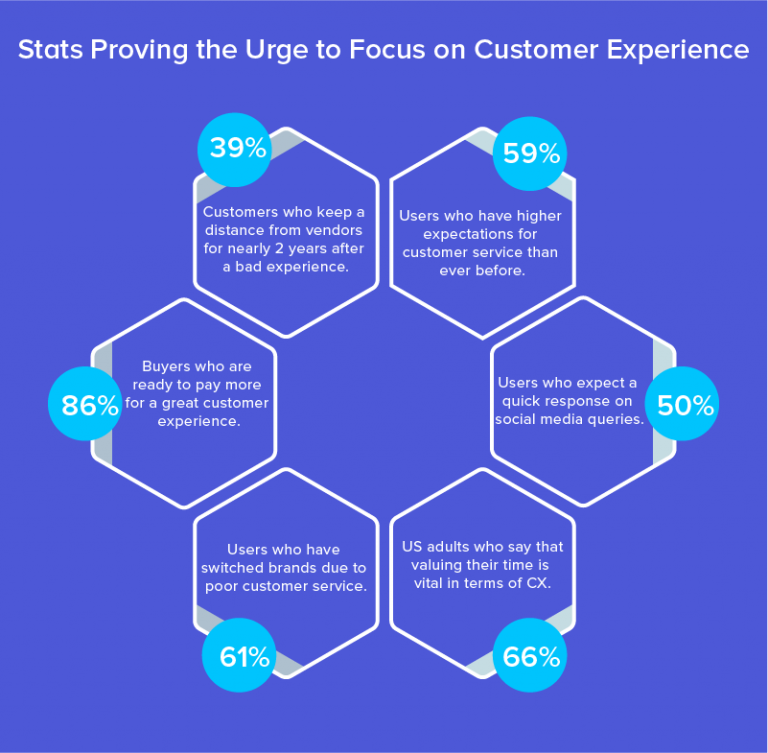
In conclusion, purpose-built AI is revolutionizing the way businesses engage with their customers, offering unprecedented levels of personalization, efficiency, and predictive insight.
By harnessing the power of AI technologies, companies can build stronger, more meaningful relationships with their customers, driving increased satisfaction, loyalty, and long-term success.
As technology continues to advance, the role of purpose-built AI in shaping customer experiences will likely become more pronounced, offering exciting possibilities for businesses aiming to stay at the forefront of their industries.
xii. Further references
Harvard Business Reviewhttps://hbr.org › 2022/03 › custome…Customer Experience in the Age of AI
TechTargethttps://www.techtarget.com › tipWill AI replace customer service reps?
FutureCIOhttps://futurecio.tech › ai-is-great-b…AI is great, but purpose-built AI is even better
KPMGhttps://kpmg.com › global-cee-2023AI and the orchestrated customer experience