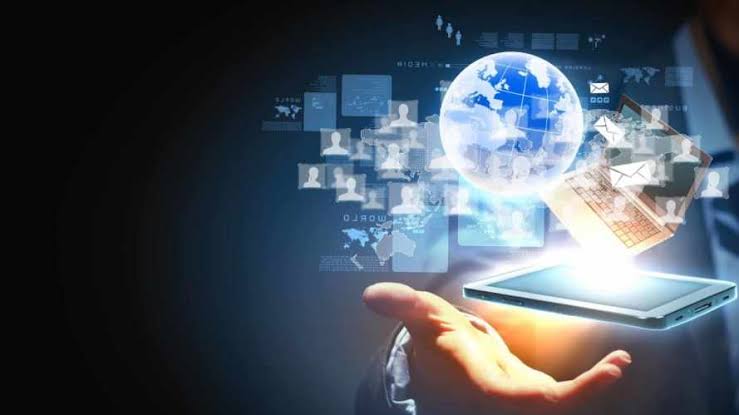
Evolution of Data Science: Proliferation and Transformation
The journey of data science from a nascent field to a cornerstone that underpins modern technological innovation embodies the transformative impact of data on society and industry.
This evolution is not only a tale of technological advancements but also of a paradigm shift in how data is perceived, analyzed, and leveraged for decision-making.
i. The Genesis and Early Years
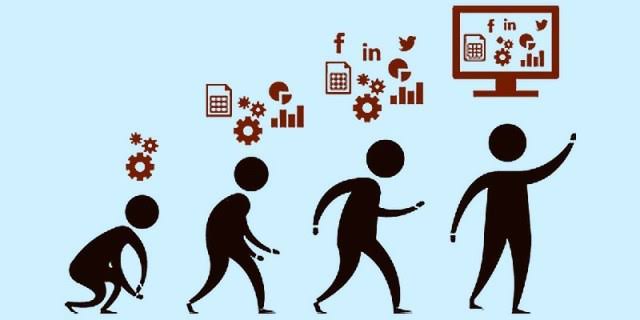
The term “data science” may have soared in popularity in recent years, yet its foundations were laid much earlier, dating back to the latter half of the 20th century.
Initially, the focus was on statistics and applied mathematics, fields that provided the tools for rudimentary data analysis. The potential of data was recognized, albeit in a limited scope, primarily in research and academic circles.
In the 1970s and 1980s, with the advent of more powerful computers and the development of relational databases, the ability to store, query, and manage data improved significantly, setting the stage for what would become known as data science.
ii. The 1990s: The Digital Explosion and the Internet Age
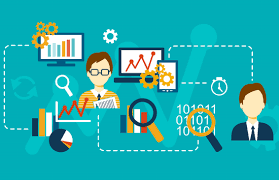
The 1990s witnessed a digital explosion, with the advent of the World Wide Web and a dramatic increase in the volume of digital data being generated.
This era introduced the term “data mining” — the process of discovering patterns in large data sets — and saw the early development of machine learning algorithms, which would become a cornerstone of modern data science. The burgeoning field aimed not just to manage or understand data, but to predict and influence future outcomes and decisions.
iii. The 2000s: Digital Revolution
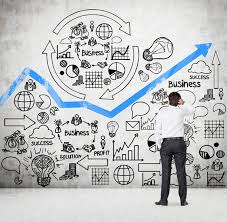
The proliferation of digital technologies in the late 20th century unleashed an explosion of data, giving rise to the era of big data.
With the advent of the internet, social media, and sensor networks, organizations found themselves inundated with vast amounts of structured and unstructured data. This deluge of data presented both challenges and opportunities, spurring the need for advanced analytical tools and techniques.
iv. 2010s to onward: The Rise of Algorithms and Machine Learning
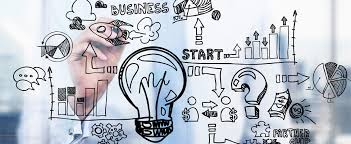
The challenge of big data was met with the rise of sophisticated algorithms and machine learning techniques, propelling data science into a new era.
Machine learning, a subset of artificial intelligence, enabled the analysis of vast datasets beyond human capability, uncovering patterns, and insights that were previously inaccessible.
This period saw not just a technological leap but a conceptual one – the shift towards predictive analytics and decision-making powered by data-driven insights.
v. Enter Data Science: Bridging the Gap
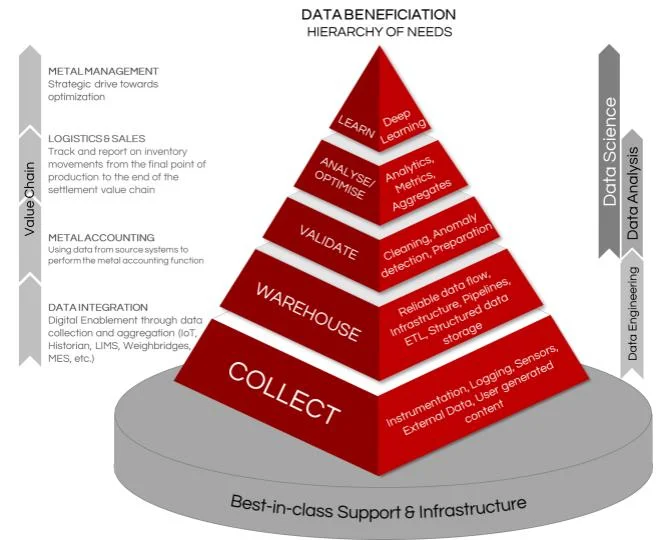
Data science emerged as the answer to the challenges posed by big data. Combining elements of statistics, computer science, and domain expertise, data scientists were equipped to extract insights from complex datasets and drive data-driven decision-making.
Techniques such as machine learning, data mining, and predictive analytics became indispensable tools for extracting value from data and gaining a competitive edge.
vi. From Descriptive to Prescriptive Analytics
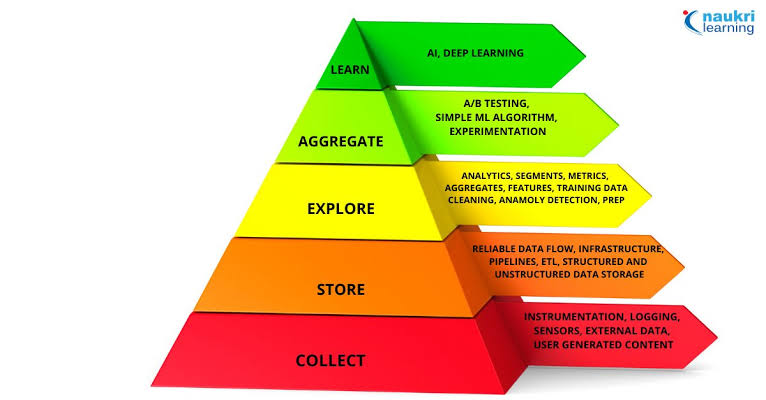
As data science matured, its focus shifted from descriptive analytics—understanding what happened in the past—to predictive and prescriptive analytics.
Predictive analytics leverages historical data to forecast future trends and outcomes, enabling organizations to anticipate customer behavior, optimize processes, and mitigate risks. Prescriptive analytics takes it a step further by providing actionable recommendations to optimize decision-making in real-time.
vii. The Era of Artificial Intelligence and Machine Learning
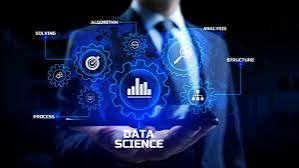
Artificial intelligence (AI) and machine learning (ML) have emerged as the cornerstone of modern data science. Powered by algorithms that can learn from data, AI and ML enable computers to perform tasks that traditionally required human intelligence.
From recommendation systems and natural language processing to image recognition and autonomous vehicles, AI and ML applications are revolutionizing industries and driving unprecedented innovation.
viii. The Democratization of Data Science
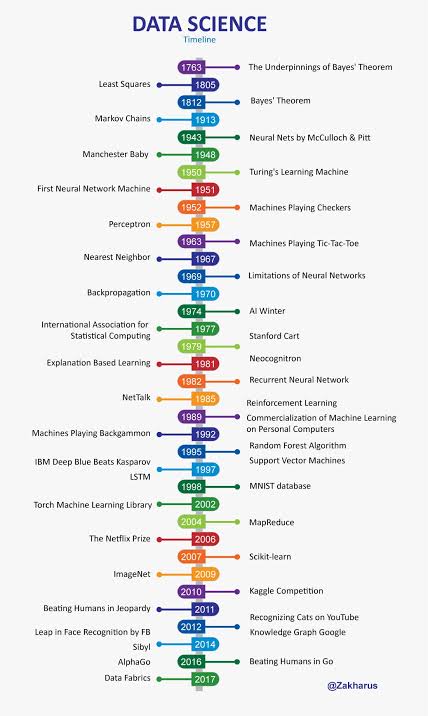
The current phase of data science evolution can be characterized by its democratization. Advanced data analysis tools and platforms have become more user-friendly and accessible, opening the doors to a wider audience beyond data scientists and statisticians.
This democratization is coupled with an emphasis on ethical AI and responsible data usage, reflecting a maturing understanding of data’s power and the importance of harnessing it wisely.
ix. Ethical Considerations and Responsible AI
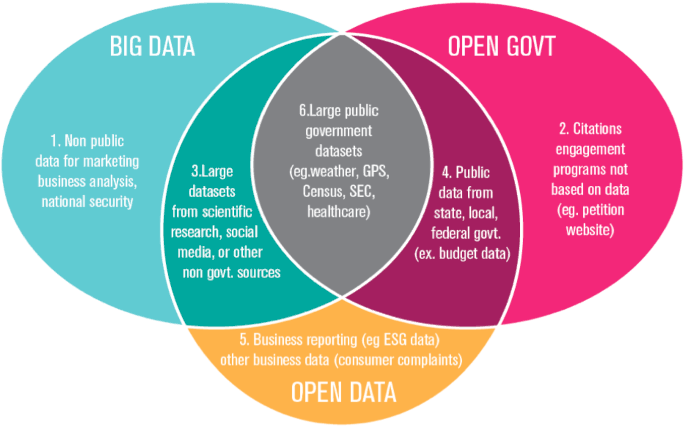
As data science continues to evolve, it is essential to address ethical considerations and ensure the responsible use of AI and ML technologies.
Concerns about data privacy, bias in algorithms, and the societal impact of AI have prompted calls for ethical frameworks and regulations to govern the use of data. Responsible AI practices prioritize fairness, transparency, and accountability, ensuring that data-driven innovations benefit society as a whole.
x. The Future of Data Science: Trends and Innovations
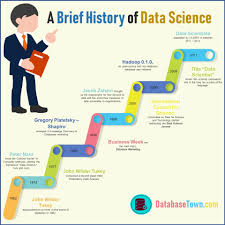
Looking ahead, the future of data science is brimming with possibilities. Emerging trends such as federated learning, edge computing, and quantum computing promise to unlock new frontiers in data analysis and AI.
The democratization of data science tools and the rise of citizen data scientists will empower individuals and organizations to harness the power of data for innovation and social good.
xi. Conclusion
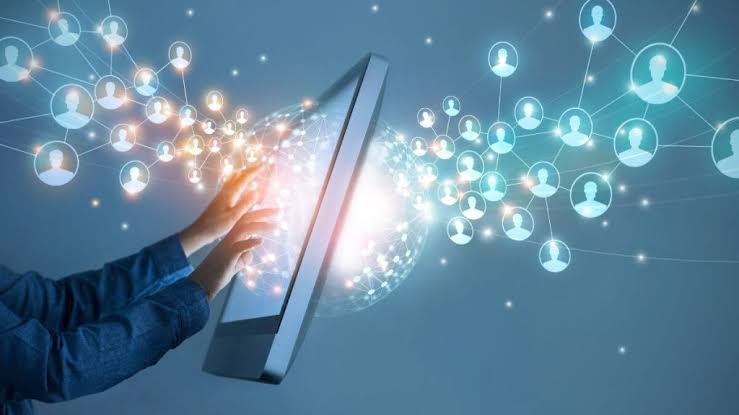
The evolution of data science from a nascent discipline to a cornerstone of modern innovation reflects the transformative power of data.
From its humble beginnings to its current state as a catalyst for innovation, data science has reshaped industries, empowered decision-makers, and unlocked new opportunities for growth.
As we continue on this journey, it is essential to embrace ethical principles and responsible practices to ensure that data-driven innovation benefits society while minimizing risks and maximizing opportunities for all.
xii. Further references
The Evolution of Data Science: Past, Present, and Future Trends
Dataquesthttps://www.dataquest.io › blogEvolution of Data Science: Growth & Innovation
PECB Insightshttps://insights.pecb.com › evoluti…Evolution of Data Science Growth and Innovation
LinkedIn · Aditya Singh Tharran6 reactions · 5 months agoThe Evolution of Data Science: Past, Present, and Future
Analytics Vidhyahttps://www.analyticsvidhya.com › i…The Evolution and Future of Data Science Innovation
Softspace Solutionshttps://softspacesolutions.com › blogEvolution of Data Science: Growth & Innovation with Python
Medium · Surya Edcater3 months agoThe Evolution of Data Science: Trends and Future Prospects | by Surya Edcater
ResearchGatehttps://www.researchgate.net › 3389…(PDF) The evolution of data science and big …
ResearchGatehttps://www.researchgate.net › 328…(PDF) The Evolution of Data Science: A New Mode of Knowledge Production
Medium · Shirley Elliott6 months agoThe Impact and Evolution of Data Science | by Shirley Elliott
Dataversityhttps://www.dataversity.net › brief-…A Brief History of Data Science
SAS Institutehttps://www.sas.com › analyticsData Scientists: Pioneers in the Evolution of Data Analysis
Institute of Datahttps://www.institutedata.com › blogExplore How Data Science Is Helping to Change the World
The World Economic Forumhttps://www3.weforum.org › …PDFData Science in the New Economy – weforum.org – The World Economic Forum
Train in Datahttps://www.blog.trainindata.com › …How Data Science is Changing the World, a Revolutionary Impact
Binarikshttps://binariks.com › BlogTop 9 Data Science Trends in 2024-2025