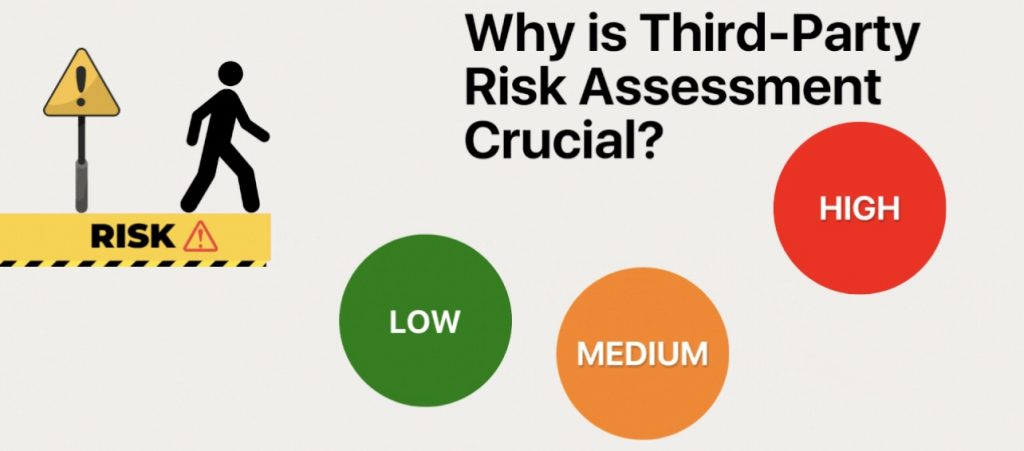
Third-Party Risk: A Crucial Element of Your GRC Program
In the increasingly interconnected landscape of modern business, organizations frequently leverage third-party vendors for a variety of services and solutions, from cloud storage and IT infrastructure to payroll and customer management systems.
While these partnerships can drive efficiency, reduce costs, and enable companies to focus on their core competencies, they also introduce third-party risks that organizations must manage.
The challenge of mitigating these risks necessitates their integration into a comprehensive Governance, Risk Management, and Compliance (GRC) program.
i. What is GRC?
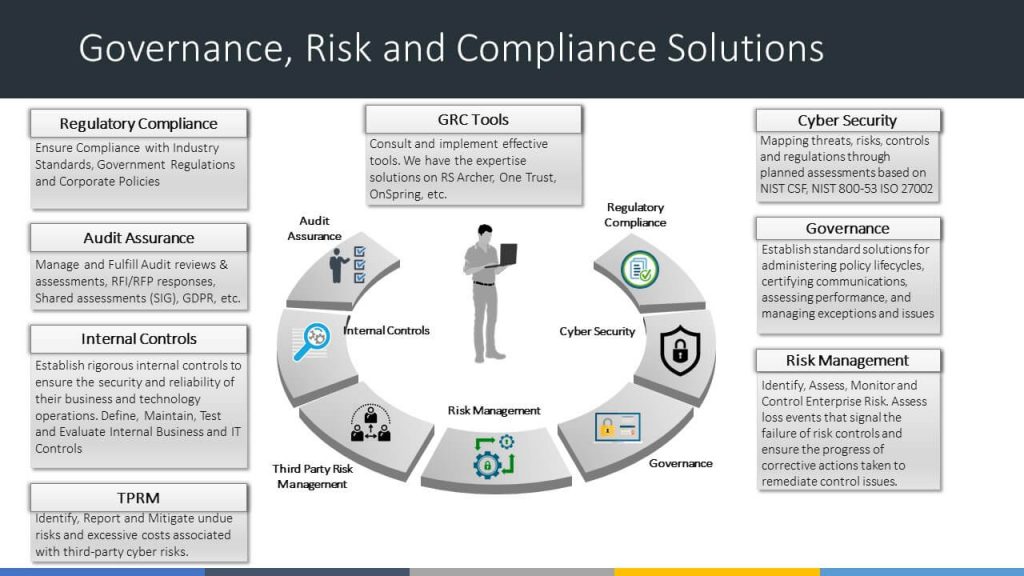
Before delving into the role of third-party risk, it’s essential to understand GRC. Governance, Risk, and Compliance encompass the policies, processes, and controls put in place by organizations to ensure they operate efficiently, ethically, and in compliance with applicable laws and regulations.
o Governance: Refers to the system of rules, processes, and structures by which an organization is directed and controlled.
o Risk Management: Involves identifying, assessing, and mitigating risks that could potentially hinder an organization’s ability to achieve its objectives.
o Compliance: Ensures that an organization adheres to relevant laws, regulations, standards, and internal policies.
ii. Why Third-Party Risk Matters
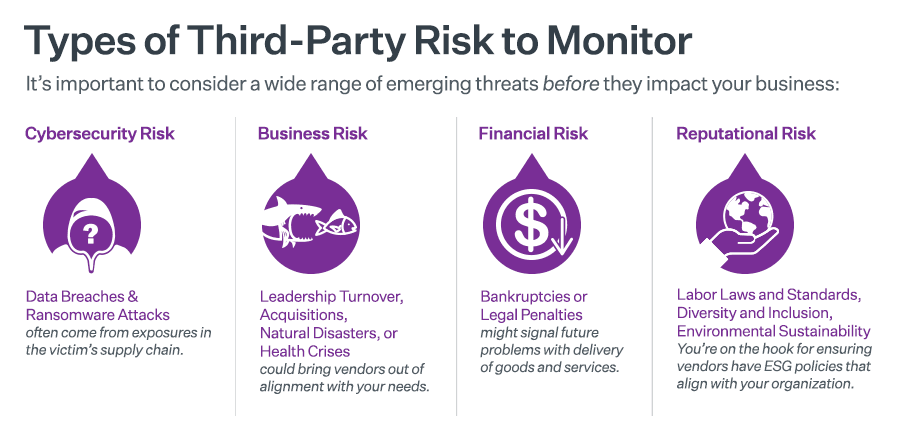
Third-party relationships can expose your organization to a variety of risks, including:
o Security breaches: Third-party vendors may have inadequate security measures, making them vulnerable to cyberattacks that could compromise your data.
o Compliance failures: Third parties may not comply with relevant regulations, putting your organization at risk of fines and reputational damage.
o Business continuity disruptions: If a third-party vendor experiences a disruption, it can impact your operations.
iii. Understanding Third-Party Risks
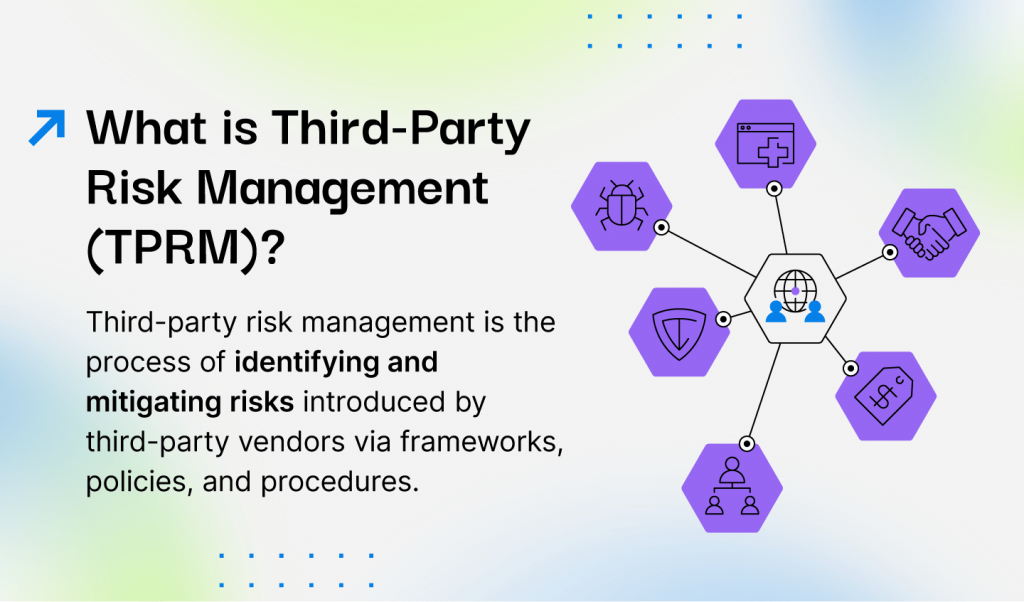
Third-party risks arise from reliance on external entities to perform or support business functions. These risks can be multifaceted, encompassing cyber threats, data privacy concerns, operational vulnerabilities, and compliance lapses.
A failure or breach in a vendor’s systems can have direct repercussions on an organization, leading to financial loss, reputational damage, and regulatory penalties.
The globalized economy and the digital nature of business operations have amplified these risks, making third-party risk management (TPRM) an essential component of any robust GRC program.
iv. Integrating TPRM into GRC
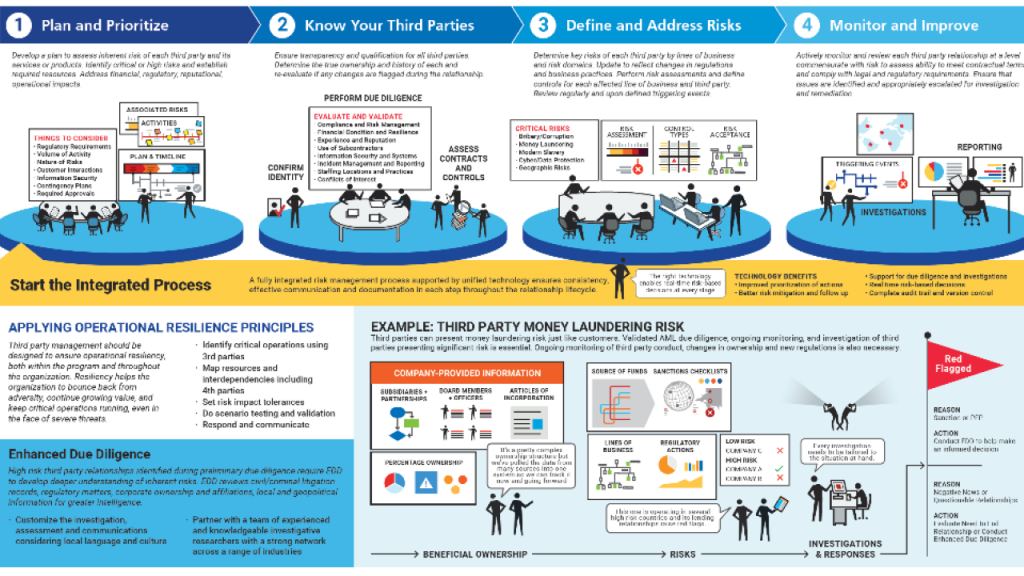
By incorporating TPRM into your GRC program, you can proactively identify, assess, and mitigate third-party risks. Here’s how:
o Vendor onboarding: Establish a process for vetting potential third parties, including risk assessments and security reviews.
o Contract management: Ensure that contracts with third parties clearly define risk expectations and responsibilities.
o Ongoing monitoring: Continuously monitor the performance of third parties and update risk assessments as needed.
v. Incorporating Risk from External Partners into Governance, Risk Management, and Compliance Frameworks
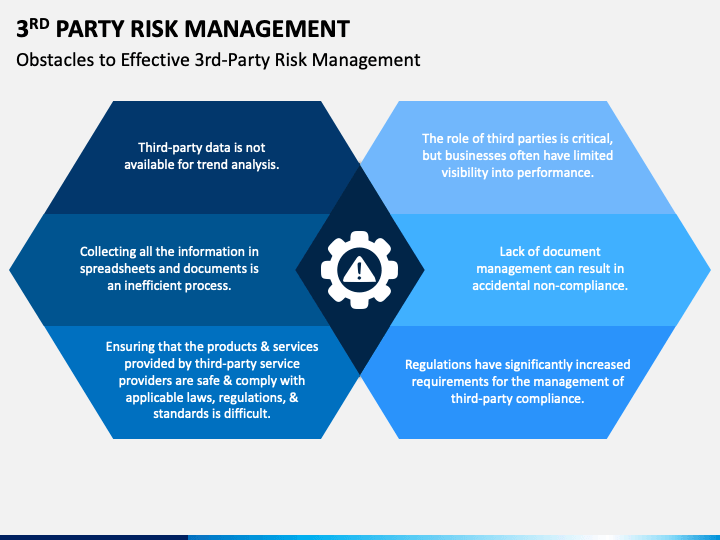
The integration of third-party risk management into your GRC program involves several key steps:
A. Risk Identification and Assessment
Start by cataloging all third parties that interact with your business processes and data. Conduct thorough risk assessments for each, considering the nature of the interaction, the sensitivity of shared data, and the third party’s security and compliance posture. This process helps prioritize risks based on their potential impact and likelihood, guiding resource allocation for mitigation efforts.
B. Due Diligence and Ongoing Monitoring
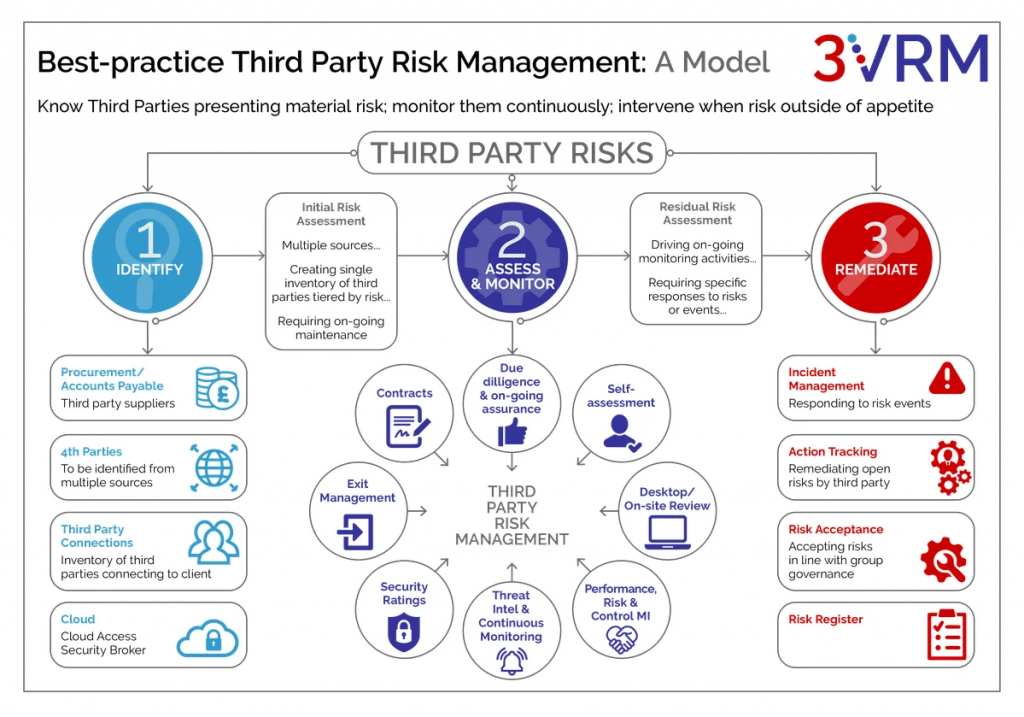
Due diligence is critical before onboarding a new third-party service provider and should be an integral part of the GRC framework. This includes evaluating the vendor’s security measures, compliance with relevant regulations (e.g., GDPR, HIPAA), and their ability to maintain service levels under adverse conditions. Ongoing monitoring is equally important to ensure that third parties continue to meet these standards throughout the duration of their contract.
C. Contract Management and Compliance
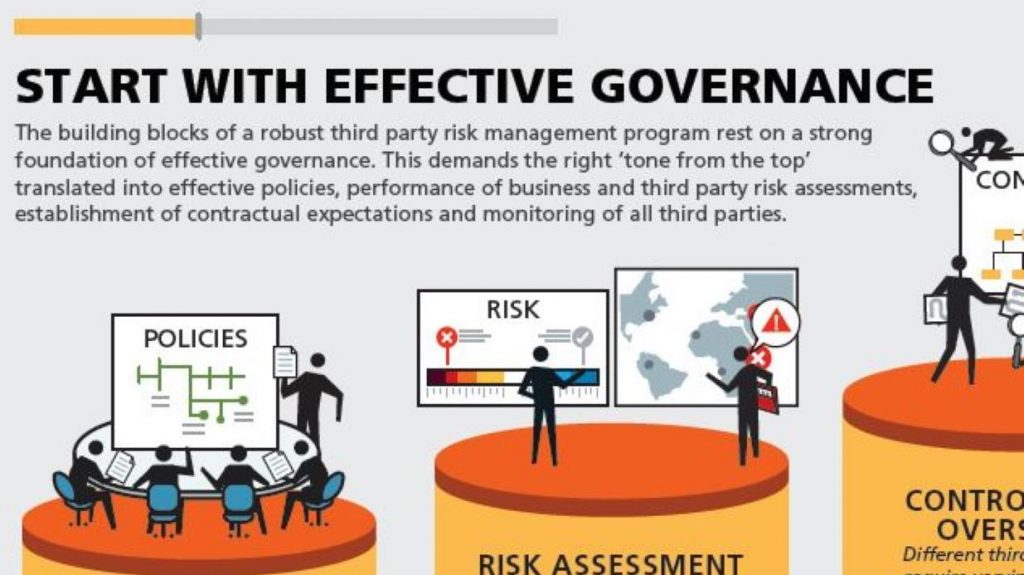
Effective contract management ensures that agreements with third parties include clauses and standards for security, compliance, and data privacy that align with your organization’s policies. This includes the right to audit the third party’s practices, data breach notification requirements, and specific levels of service. Compliance management ensures that third-party practices align with regulatory requirements and industry standards, mitigating legal and regulatory risks.
D. Ongoing Monitoring and Oversight
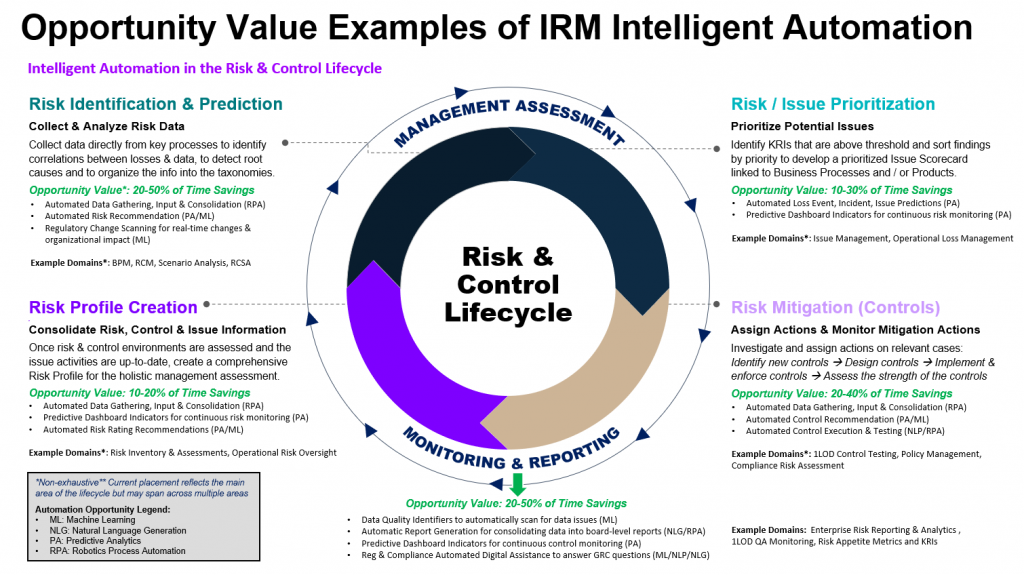
o Continuous Monitoring: Implement processes to monitor third-party activities, performance, and compliance with contractual obligations and regulatory requirements.
o Regular Assessments: Conduct periodic risk assessments and audits to ensure ongoing adherence to established standards and identify emerging risks.
E. Incident Management and Business Continuity Planning

Prepare for potential incidents involving third parties by establishing processes for swift action and communication. Your GRC program should include third-party risks in its incident response and business continuity plans, ensuring that there are procedures in place to minimize downtime and mitigate the impact of any breaches or failures.
F. Education and Awareness
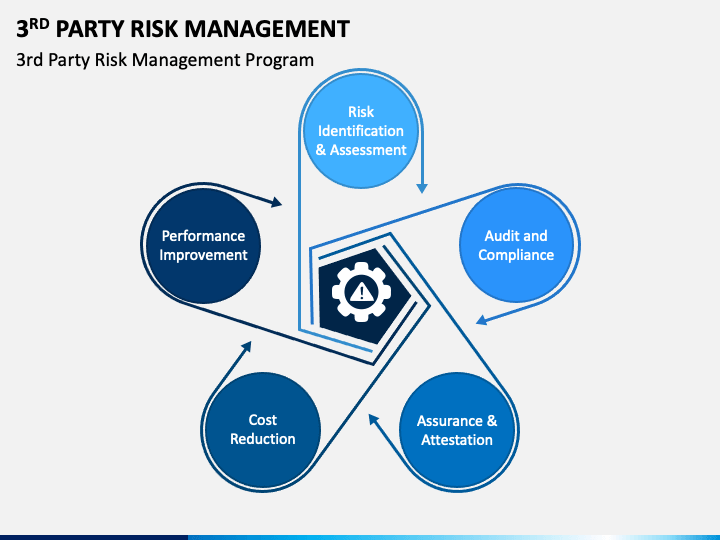
Educate your organization’s stakeholders about the risks associated with third parties and the importance of due diligence and ongoing monitoring. A culture of risk awareness can drive more responsible decision-making and risk management practices across all levels of the organization.
vi. Challenges and Considerations
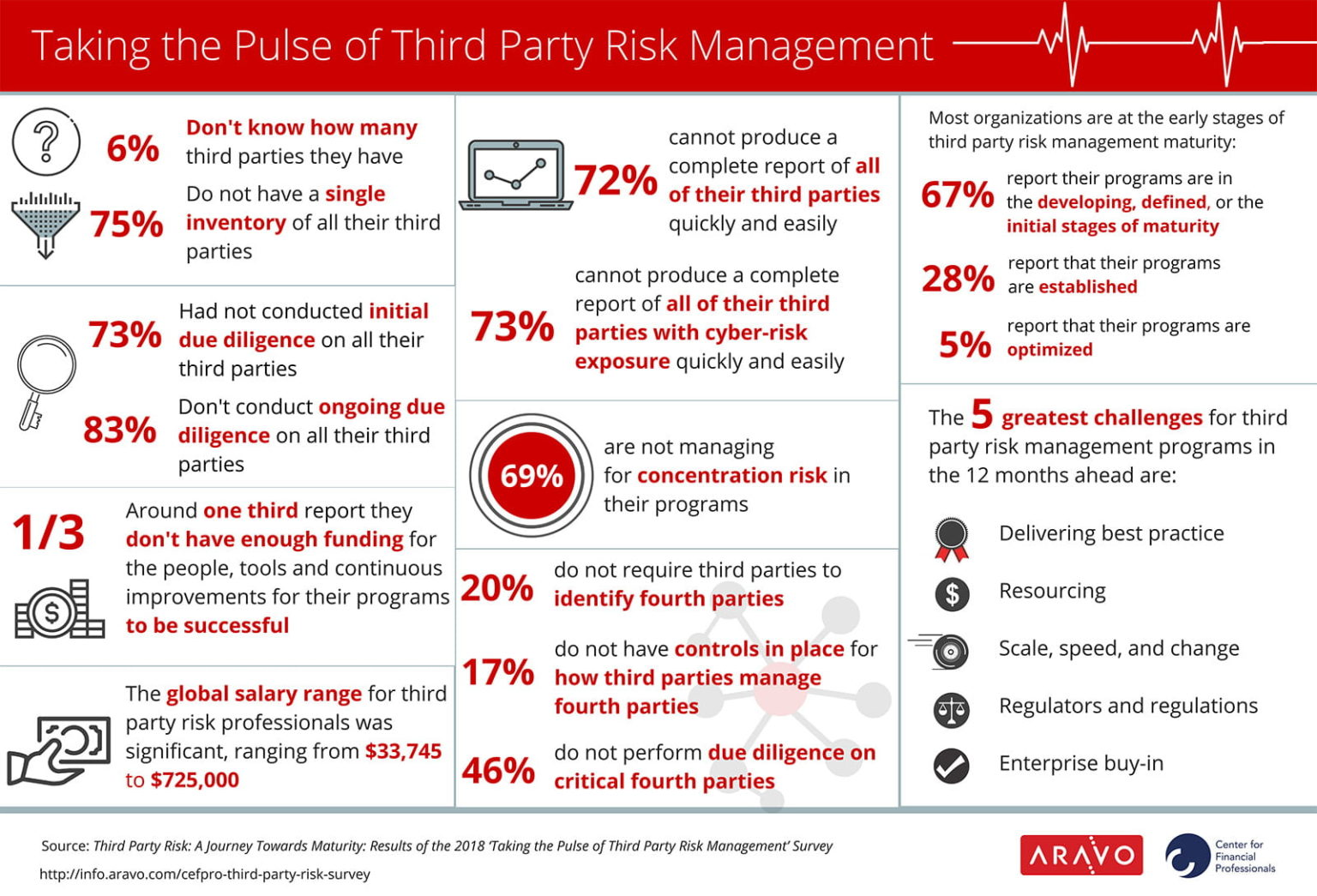
Integrating third-party risk into your GRC program involves navigating challenges such as the complexity of third-party relationships, the dynamic nature of risk, and the necessity of balancing risk management with business innovation. A successful program requires a combination of thorough assessment, continuous monitoring, and flexible strategies that can adapt to new threats and business needs.
vii. Strategies for Successful Integration
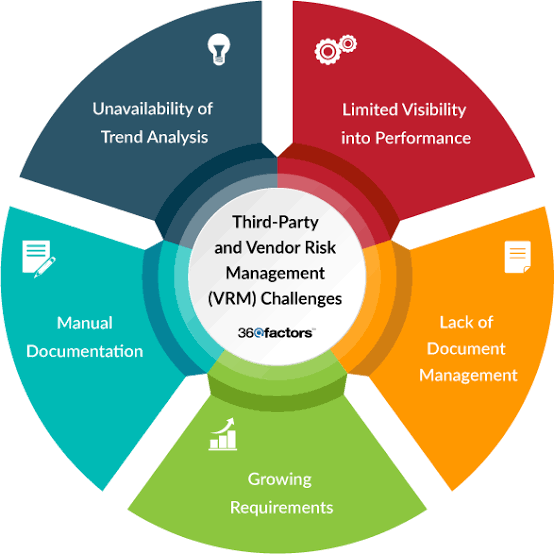
o Centralize Third-Party Risk Management: Establish a unified program that oversees all third-party risks, ensuring consistency and eliminating silos.
o Leverage Technology: Utilize GRC technology platforms that incorporate third-party risk management capabilities. This can streamline assessments, monitoring, and reporting processes.
o Build Cross-Functional Teams: Create a cross-disciplinary team involving members from legal, procurement, IT, compliance, and other relevant departments to address multifaceted third-party risks.
o Educate and Train: Foster a culture of risk awareness across the organization, including understanding the significance of third-party risks and the role of employees in mitigating them.
o Establish Strong Contracts and SLAs: Define clear expectations, responsibilities, and consequences related to security, compliance, and performance in all third-party contracts and Service Level Agreements (SLAs).
viii. Benefits of Effective TPRM
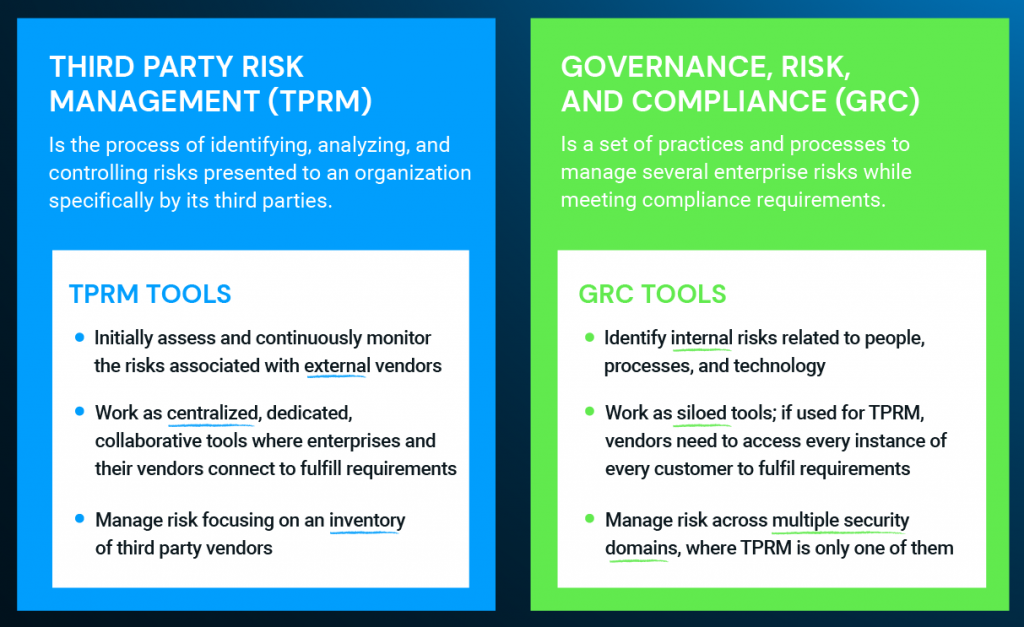
A well-integrated TPRM program can bring significant benefits to your organization:
o Reduced risk of security breaches and data loss
o Enhanced compliance posture
o Improved operational resilience
o Stronger vendor relationships
ix. Conclusion
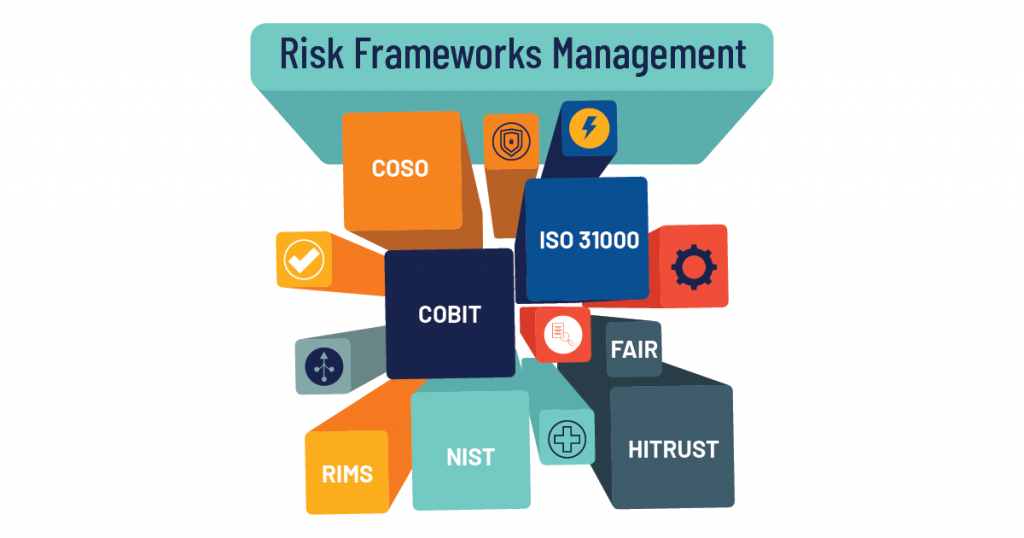
Incorporating third-party risk into your GRC program is not a one-time activity but an ongoing process that evolves with the threat landscape, technological advances, and regulatory changes.
As organizations continue to extend their operations through a network of third-party relationships, the importance of a holistic approach to third-party risk in GRC strategies cannot be overstated.
By effectively embedding third-party risk considerations into governance, risk management, and compliance activities, organizations can protect their assets, reputation, and ultimately, their success in the market.
x. Further references
Third-Party Risk Management Considerations for Your GRC Strategy
Centraleyeshttps://www.centraleyes.com › key…Understanding the Key Differences Between TPRM and GRC
Secureframehttps://secureframe.com › hub › grcWhat Is Third-Party Risk Management + Policy
GRF CPAs & Advisorshttps://www.grfcpa.com › resourceA Guide to Third Party Risk Management – GRF …
Bitsighthttps://www.bitsight.com › blog › u…What is TPRM? (Guide to Third Party Risk Management)
LinkedIn · Priyanka R8 months agoBest Practices for Managing Third-Party Risk in a GRC Program
ISACAhttps://www.isaca.org › industry-newsGRC Programming: The Third-Party Security Web