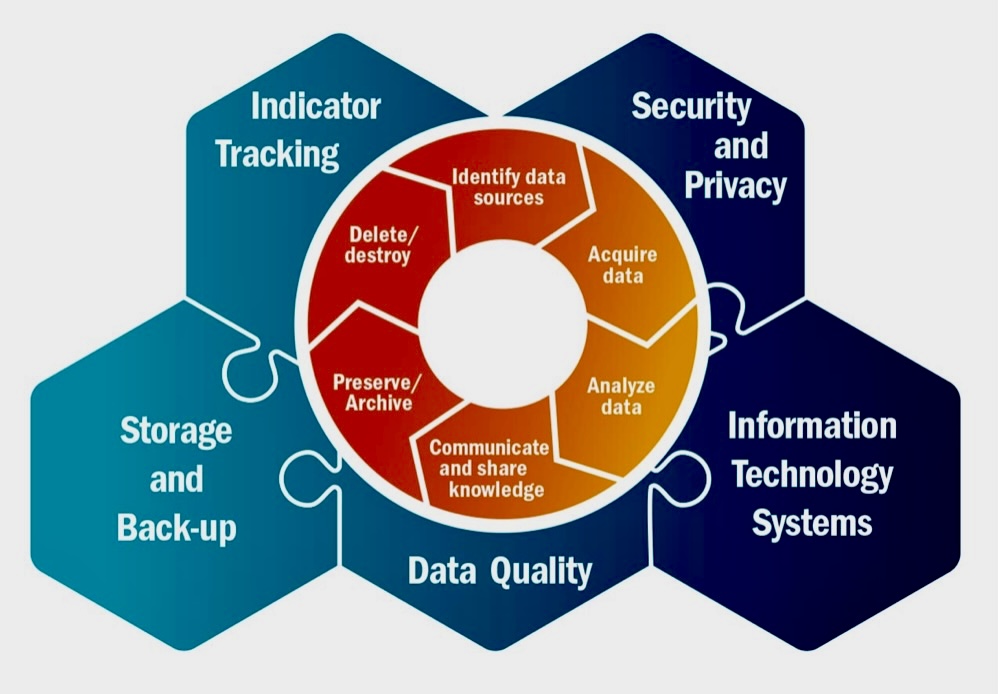
Data Provenance, the ability to trace and verify the origin of data, its movement, and its processing history, is valuable in several use cases.
Here are some of the most prominent verticals:
A. Agriculture Sector: Farmers, suppliers, and customers can use data provenance to trace a product’s origin and journey. This activates a more transparent food supply chain and supports the production of fair trade, organic and sustainably sourced products.
B. Art Industry: In this field, data provenance helps authenticate and trace the origins of artwork. This validates authenticity, ownership, and helps prevent art forgery.
C. Business Analytics: Provenance allows businesses to trace the origin of the data behind their business intelligence insights, which adds an additional level of confidence and credibility to their decision-making process.
D. Cybersecurity: Organizations use data provenance to keep track of changes made to their data. By knowing the source and history of a file, firms can better detect unauthorized data access or manipulation.
E. Data Governance: Organizations employ data provenance in their data governance strategy to understand their data sources, transformations, and users better, thereby ensuring high data quality.
F. Digital Forensics: Provenance assists in tracking the source and movement of digital information that can help in crime investigations and fraud detection.
G. Education Sector: Universities and education providers can use data provenance to authenticate academic credentials, thereby reducing instances of qualification fraud.
H. Energy Sector: Energy companies use data provenance to optimize their energy distribution, track energy consumption, and implement better energy-saving solutions.
I. Finance and Banking: For regulatory and auditing purposes, banks and financial institutions should trace all the financial transactions. Provenance ensures transactions are valid and helps to detect fraudulent activities.
J. Government and Public Services: Governments can use data provenance to authenticate and trace documents, improving public service transparency and efficiency. It’s also useful in fraud detection and prevention.
K. Healthcare: Medical records often pass through various departments, clinics, or hospitals. Data provenance ensures the traceability of patient records, prescriptions, treatments, and diagnosis histories, essential for patient safety and care.
L. Insurance: Companies use data provenance for claims management and fraud detection. Insurers can trace and verify the origin of the claim data, making it easier to identify potential fraud.
M. Journalism and Media: With fake news on the rise, data provenance can help verify the origin of information, increasing trust in published content.
O. Pharmaceutical Industry: Here, data provenance is used to validate the origins of medication and verify its journey through the supply chain. This can prevent counterfeit drug distribution and ensure patient safety.
P. Scientific research: Data provenance plays a crucial role in experimental sciences where researchers need to track the origin and transformation of the data throughout their experiments, facilitating replication and validation of the results.
Q. Supply Chain Management: In industries like food, fashion, and manufacturing, data provenance helps map product origin and journey, ensuring authenticity, sustainability, and regulatory compliance.
R. Technology Industry: Technology companies use data provenance to improve the performance and reliability of their products and services.
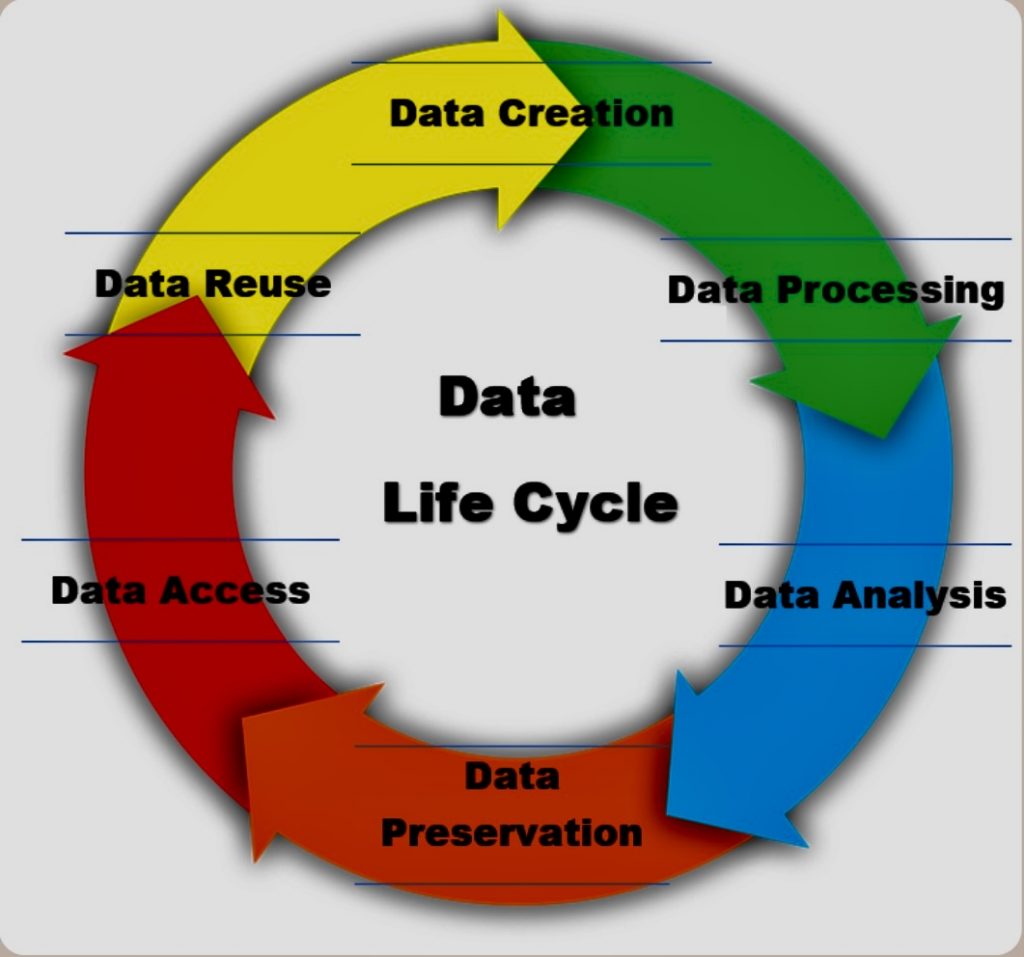
Understanding the origins and transformations of data is vital in an era where data-driven decision making is increasingly common. Using data provenance, organizations can ensure their data is accurate, consistent, and reliable.
In addition to these specific use cases, data provenance can be used to improve a variety of data-driven processes, such as data governance, data quality management, and data security.
Here are some examples of how data provenance is being used in practice:
A. Auditing and Accountability: Facilitating auditing processes by allowing organizations to trace the flow of data and understand who accessed or modified it. This enhances accountability and helps in identifying potential security breaches or unauthorized access.
B. Blockchain and Smart Contracts: Supporting blockchain applications and smart contracts by providing a transparent record of data transactions. This enhances the trustworthiness and reliability of blockchain-based systems.
C. Business Process Optimization: Optimizing business processes by analyzing the data provenance to identify bottlenecks, inefficiencies, or areas for improvement. This contributes to overall process optimization and efficiency gains.
D. Comprehensive Analytics: Enabling data scientists and analysts to understand the context and history of the data they are working with. This supports more accurate and informed analyses, leading to better business insights.
E. Data Governance: Strengthening data governance initiatives by establishing a comprehensive understanding of data lineage, ownership, and usage within an organization. This ensures that data is managed responsibly and in accordance with governance policies.
F. Data Integration and Transformation: Facilitating data integration processes by enabling a detailed understanding of how different datasets are transformed and integrated. This is valuable for maintaining data consistency and integrity across diverse sources.
G. Data Quality Management: Improving data quality by identifying the source of errors, inconsistencies, or inaccuracies in datasets. Data provenance enables organizations to trace back to the origin of issues and implement corrective measures.
H. Digital Forensics: Aiding digital forensics investigations by providing a historical record of data changes and access. This is critical for analyzing security incidents, identifying the extent of a breach, and determining the cause.
I. Fraud Detection and Prevention: Enhancing fraud detection capabilities by tracking the history of data transformations and identifying anomalous patterns or changes in the data that may indicate fraudulent activities.
J. Machine Learning Model Transparency: Enhancing transparency in machine learning models by tracking the provenance of training data, feature engineering, and model configurations. This is particularly important for model interpretability and fairness.
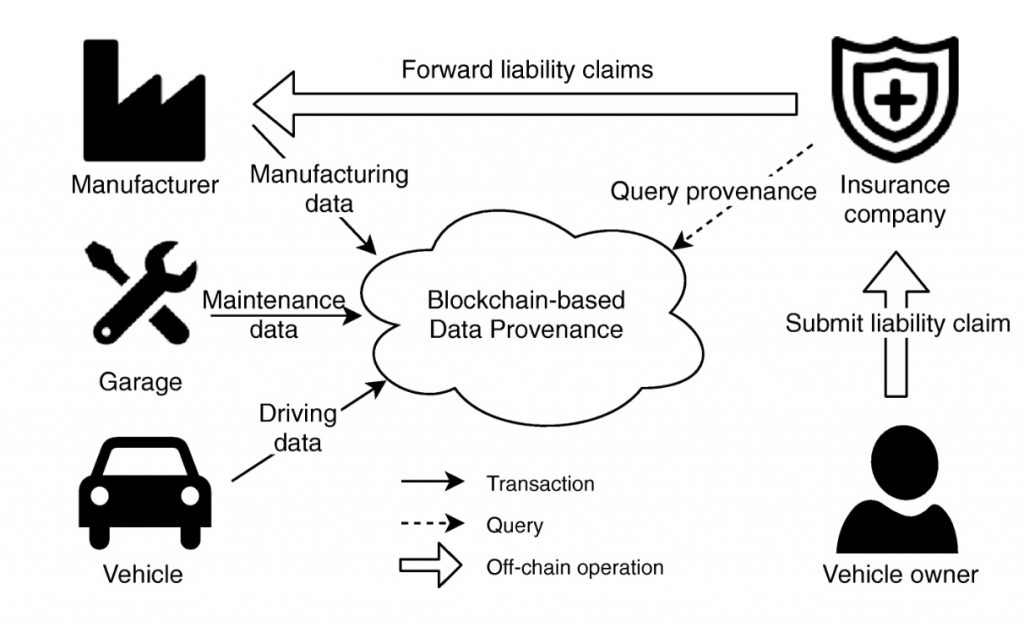
K. Regulatory Compliance: Demonstrating compliance with data protection regulations, such as GDPR or HIPAA, by providing a clear lineage of how and where personal data is collected, processed, and stored.
L. Risk Management: Improving risk management by providing a clear view of the data used in decision-making processes. Organizations can assess the reliability of data and understand potential risks associated with certain datasets.
M. Scientific Research and Reproducibility: Supporting reproducibility in scientific research by documenting the origin and processing steps of data used in experiments. This helps other researchers validate results and build upon previous studies.
N. Supply Chain Visibility: Providing transparency and visibility into the entire supply chain by tracking the origin and movement of products and related data. This is particularly valuable in industries like food and pharmaceuticals for ensuring product safety and authenticity.
O. Transparency: Data provenance can help to increase transparency and trust in data-driven decision-making. By understanding the origin and history of data, organizations can better explain their decisions and build trust with stakeholders.
These functions demonstrate the diverse applications of data provenance across various industries and scenarios, emphasizing its role in ensuring data reliability, compliance, and informed decision-making.
As data becomes increasingly important, data provenance is becoming essential for organizations of all sizes. By tracking the origin, lineage, and history of data, organizations can improve data quality, compliance, transparency, and risk management.
https://link.springer.com/chapter/10.1007/978-3-030-52829-4_12