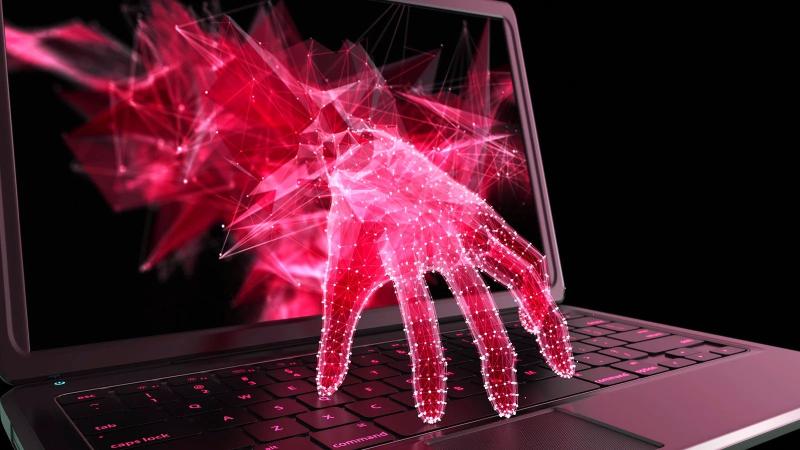
Alarming Rise: Data-Stealing Malware Infections Surge 700% Since 2020
In an age where digital connectivity underpins nearly every aspect of personal and professional life, the alarming surge in devices infected with data-stealing malware presents a critical challenge.
A recent report by Kaspersky Digital Footprint Intelligence paints a concerning picture: the number of devices infected with data-stealing malware has skyrocketed by a staggering 700% since 2020.
This alarming trend highlights the ever-evolving threat landscape and the urgency for increased cybersecurity vigilance.
This staggering rise not only underscores the growing complexity and aggression of cyber threats but also highlights vulnerabilities in current security measures.
i. What is Data-Stealing Malware?
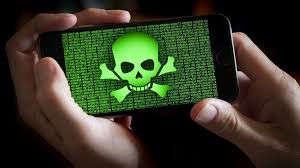
Data-stealing malware, also known as info-stealers, are malicious programs designed to infiltrate devices and steal sensitive information. This information can include login credentials, credit card details, personal data, and even corporate secrets. Unlike ransomware, which encrypts data and demands a ransom for its release, infostealers operate silently in the background, exfiltrating data without the user’s knowledge.
ii. The Surge in Data-Stealing Malware
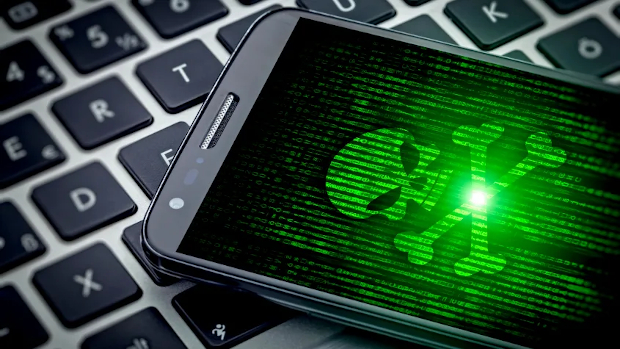
Data-stealing malware is a type of malicious software designed to intercept and exfiltrate sensitive information from infected devices. This could include login credentials, financial data, personal identification information, and intellectual property. The 700% increase since 2020 can be attributed to several factors:
A. Increased Remote Work: The COVID-19 pandemic forced many organizations to rapidly shift to remote work, often without adequate cybersecurity measures. This transition created new opportunities for cybercriminals to exploit vulnerabilities in home networks and personal devices.
B. Sophisticated Attack Techniques: Cybercriminals have developed more sophisticated malware that can bypass traditional security measures. Techniques such as phishing, spear-phishing, and the use of ransomware have become more prevalent and effective.
C. Greater Connectivity: The proliferation of Internet of Things (IoT) devices, along with increased reliance on cloud services, has expanded the attack surface for cybercriminals. Each connected device represents a potential entry point for malware.
D. Increased Reliance on Digital Tools: The COVID-19 pandemic accelerated the shift towards remote work and online transactions. This creates a larger pool of potential targets for cybercriminals.
E. Exploiting New Technologies: Cybercriminals are quick to adapt and exploit vulnerabilities in emerging technologies like cloud computing and the Internet of Things (IoT).
F. Rise of Ransomware-as-a-Service (RaaS): The growing popularity of RaaS models makes it easier for even less-skilled attackers to launch sophisticated cyberattacks, including deploying data-stealers.
iii. Consequences of Infected Devices
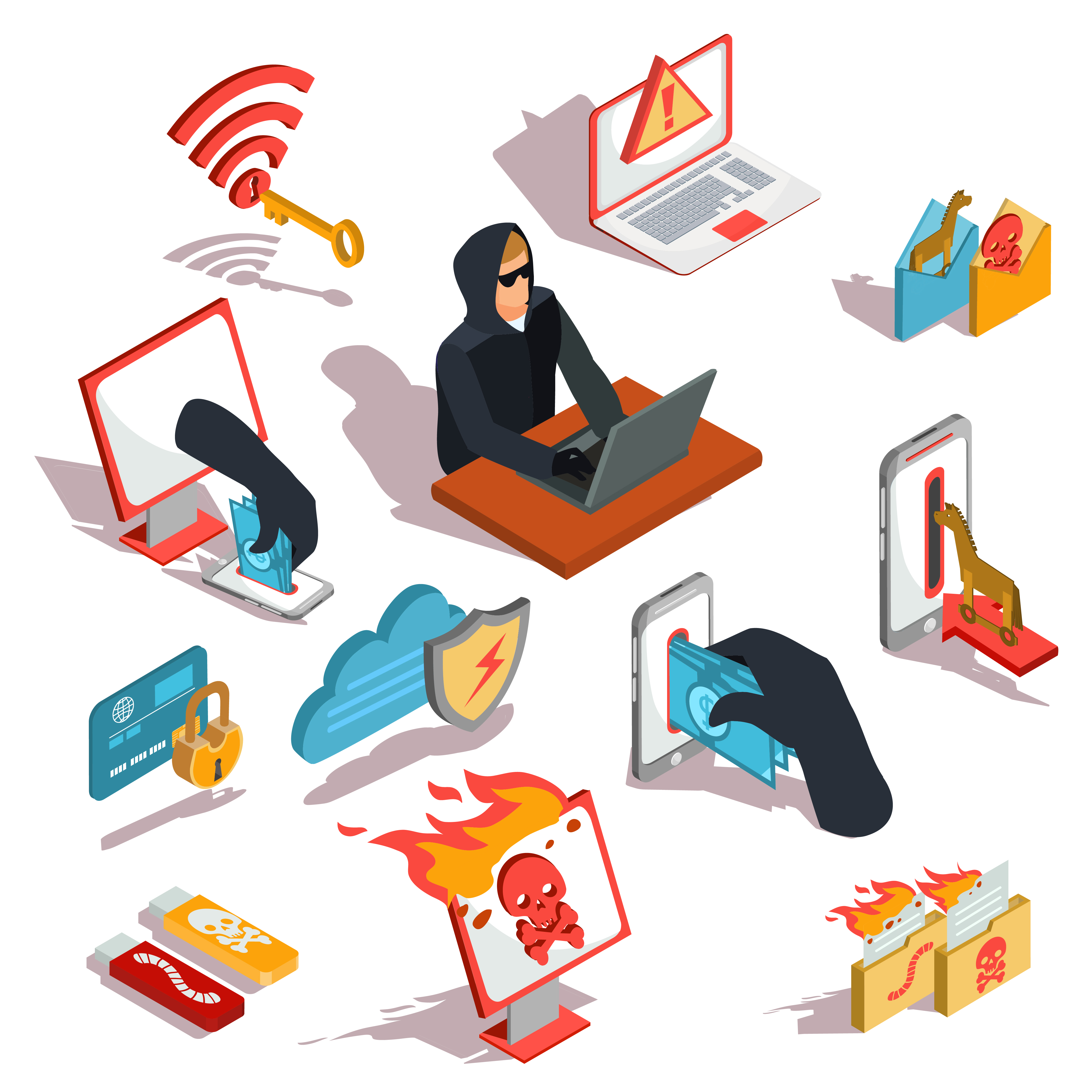
The ramifications of a device being infected with data-stealing malware are severe and wide-ranging. For individuals, it can lead to identity theft, financial loss, and privacy invasions. For businesses, the consequences can be even more dire, including:
o Financial Losses: Victims of data theft often suffer significant financial losses. Personal banking information and credit card details can be exploited, leading to unauthorized transactions and financial fraud.
o Reputational Damage: Loss of customer trust and potential loss of business due to data breaches.
o Identity Theft: Stolen personal information can be used to commit identity theft, resulting in long-term damage to victims’ credit scores and personal reputations.
o Corporate Espionage: For businesses, data-stealing malware can lead to the theft of sensitive corporate information, including trade secrets, intellectual property, and confidential client data. This can result in competitive disadvantages and legal liabilities.
o Legal Consequences: Violations of data protection regulations can result in heavy fines and legal action.
o Operational Disruption: Malware infections can disrupt business operations, leading to downtime, reduced productivity, and increased recovery costs. In some cases, the damage can be so severe that it threatens the survival of the business.
iv. The Scope of the Threat
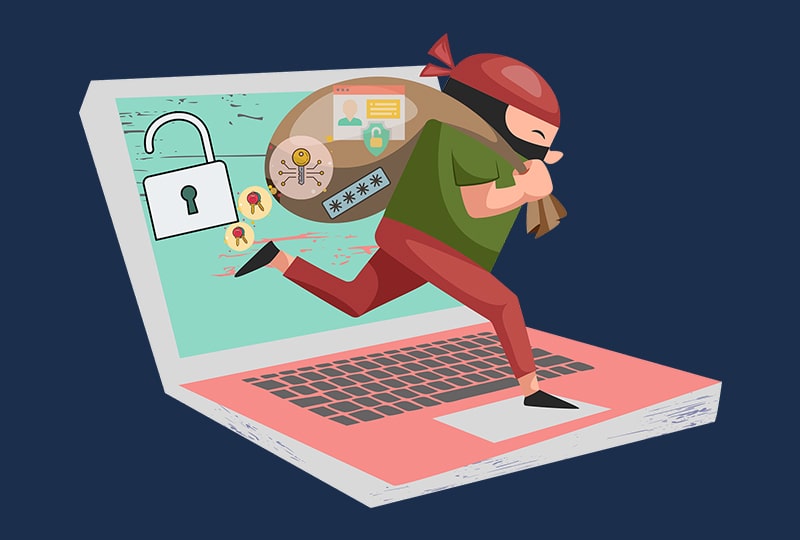
The Kaspersky report reveals that nearly 10 million devices were compromised by data-stealing malware in 2023 alone. Cybercriminals are estimated to have stolen an average of 50.9 credentials per infected device, highlighting the vast amount of sensitive data at risk. These stolen credentials can be used for a variety of criminal activities, including identity theft, financial fraud, and even corporate espionage.
v. Defensive Measures
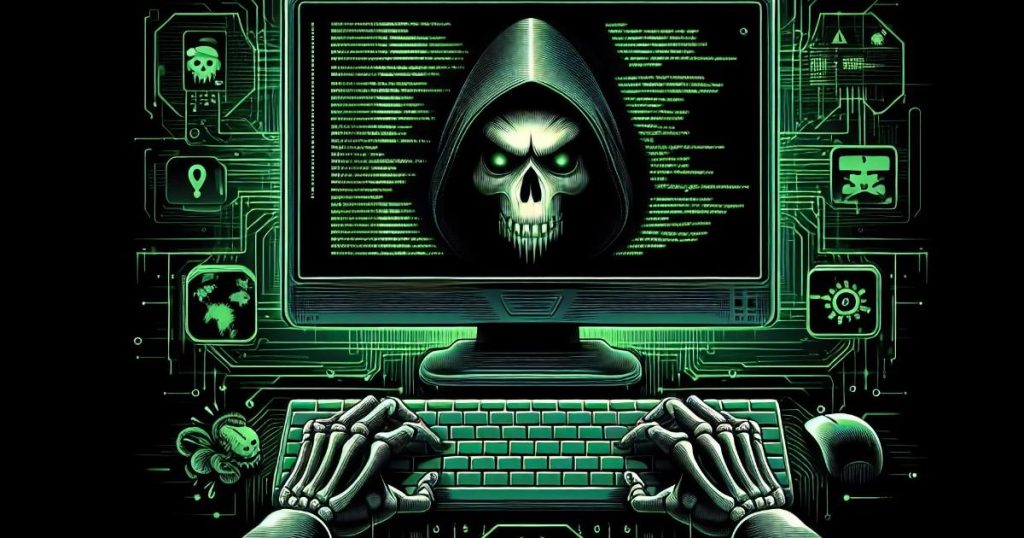
In response to the uptick in data-stealing malware, individuals and organizations need to adopt comprehensive cybersecurity strategies:
A. Regular Software Updates: Ensuring all software, including operating systems and applications, are up to date with the latest security patches.
B. Strong Authentication: Implementing multi-factor authentication (MFA) to make it more difficult for unauthorized users to access sensitive information.
C. Employee Training: Educating employees about phishing and other social engineering attacks to reduce the likelihood of successful intrusions.
D. Advanced Security Solutions: Utilizing advanced security solutions such as endpoint protection, intrusion detection systems, and encryption to safeguard data.
E. Regular Backups: Maintaining regular backups of important data to mitigate the impact of a potential ransomware attack.
F. Zero Trust Architecture: Adopting a Zero Trust approach to network security, which assumes that threats could be internal or external, can help protect sensitive data by enforcing strict access controls.
G. Data Encryption: Encrypting sensitive data ensures that even if it is stolen, it cannot be easily accessed or used by cybercriminals.
H. Network Security: Utilizing firewalls, intrusion detection systems, and secure network architectures can help protect against malware infiltration.
vi. Protecting Yourself from Data-Stealing Malware
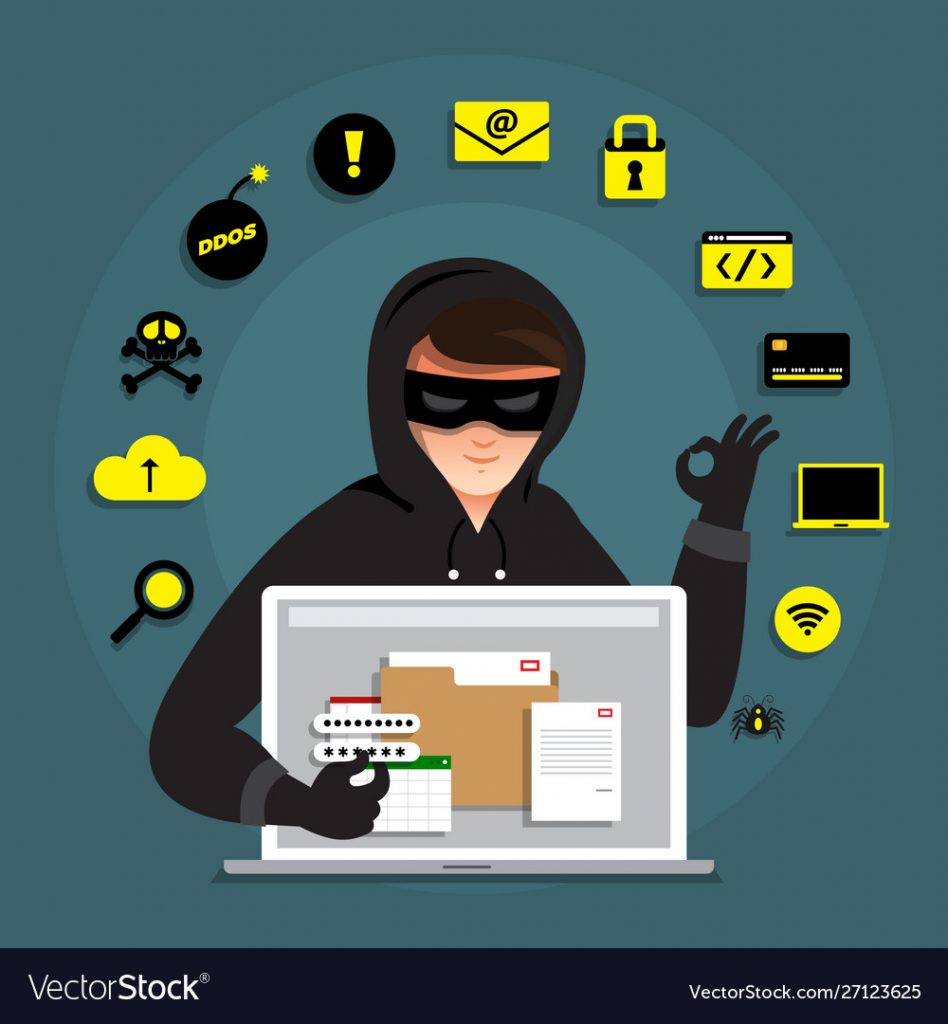
While the rise in data-stealing malware is concerning, there are steps you can take to protect yourself:
o Install Security Software: Use a reputable antivirus and anti-malware program on all your devices, and keep them updated with the latest security patches.
o Be Wary of Phishing Attempts: Phishing emails and websites are a common way for cybercriminals to distribute malware. Be cautious of suspicious links and attachments.
o Enable Two-Factor Authentication: Two-factor authentication adds an extra layer of security to your online accounts, making it more difficult for attackers to gain access even if they steal your password.
o Practice Strong Password Hygiene: Use unique and strong passwords for all your online accounts, and avoid using the same password for multiple accounts.
o Be Mindful of Downloads: Only download software and applications from trusted sources.
vii. The Road Ahead: A Collective Effort
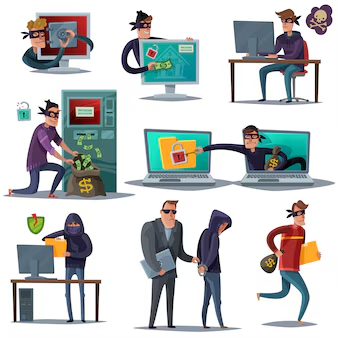
The 700% increase in devices infected with data-stealing malware since 2020 serves as a stark reminder of the evolving threat landscape.
Combating the growing threat of data-stealing malware requires a collective effort. Individuals, organizations, and cybersecurity firms need to work together to raise awareness, develop robust defenses, and foster a culture of digital security.
viii. Conclusion
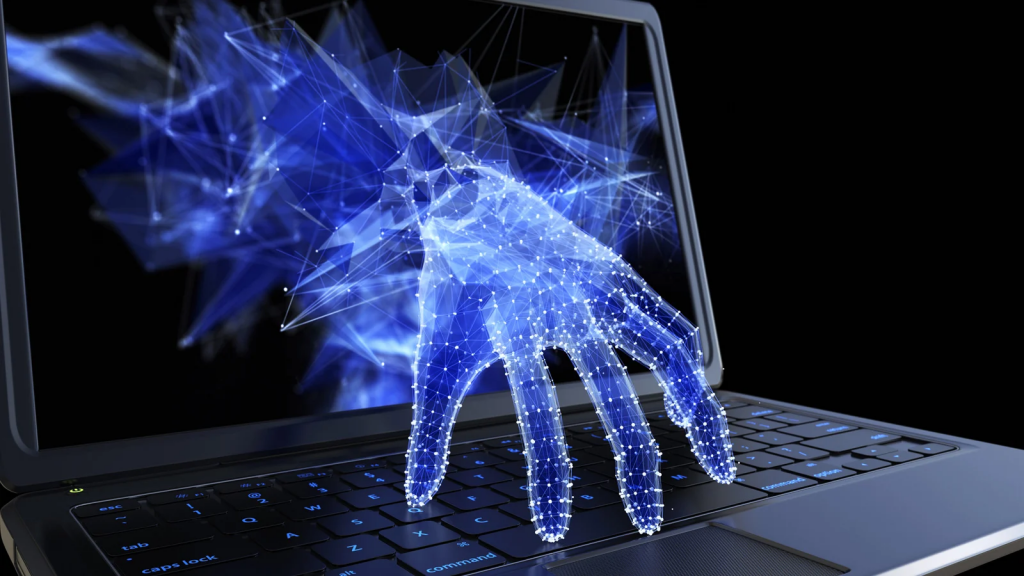
In conclusion, while the surge in data-stealing malware is concerning, it also provides an opportunity for increased awareness and improvement in cybersecurity practices. By adopting robust defensive measures and staying educated on emerging threats, we can collectively work towards a safer digital future.
ix. Further references
Devices Infected With Data-Stealing Malware Increased by 7 Times Since 2020
The Economic Timeshttps://m.economictimes.com › techmalware attack: Number of devices hit by data …