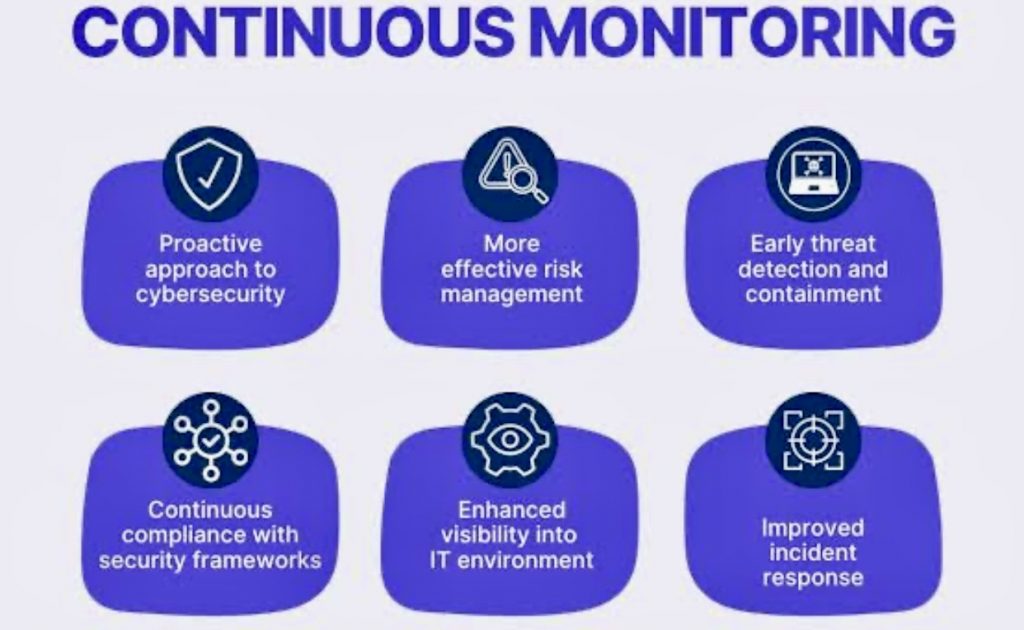
Embracing continuous monitoring is a crucial strategy for organizations to protect against escalating data breach risks. In our current digital landscape, where cyber threats are continually evolving, businesses can no longer rely solely on periodic security assessments.
i. What is continuous monitoring?
Continuous monitoring is the practice of continuously collecting and analyzing data from a variety of sources to identify and respond to threats. This data can include information from network traffic, endpoint devices, and applications. Continuous monitoring can be used to detect a wide range of threats, including:
o Malware
o Phishing attacks
o Data leaks
o Insider threats
o Denial-of-service attacks
Continuous monitoring provides real-time analysis of security controls and system configurations to identify vulnerabilities and address them promptly.
ii. Here’s why continuous monitoring can act as a shield against escalating data breach risks:
A. Real-Time Threat Detection: Continuous monitoring allows organizations to detect and respond to security threats in real-time. By constantly analyzing network activities and system logs, any unusual or suspicious behavior can be identified promptly.
B. Early Incident Identification: With continuous monitoring, organizations can identify security incidents at their early stages. This early detection enables swift response measures, minimizing the potential impact of a data breach.
C. Active Response: Continuous monitoring provides the data necessary to make informed security decisions and respond immediately to potential issues, reducing the time for attackers to exploit vulnerabilities.
D. Reduced Dwell Time: Dwell time, the duration a threat remains undetected in a network, is a critical factor in the severity of a data breach. Continuous monitoring helps reduce dwell time by quickly identifying and mitigating threats before they can escalate.
E. Proactive Risk Management: Continuous monitoring is a proactive approach to risk management. It allows organizations to identify vulnerabilities, misconfigurations, or potential weaknesses in real-time, enabling timely remediation efforts.
F. Insider Threat Detection: Monitoring user activities and access patterns helps in detecting insider threats. Continuous monitoring can identify unusual user behavior or unauthorized access, whether intentional or unintentional.
G. Compliance Verification: Many regulatory standards require continuous monitoring to ensure compliance. Real-time tracking allows companies to maintain and demonstrate compliance more easily and effectively.
H. Compliance Adherence: By embracing continuous monitoring, organizations can ensure adherence to data protection regulations and industry-specific requirements.
I. Modern Threat Management: With advanced threats like zero-day exploits, businesses need a continuous monitoring system that can keep up with emerging threat vectors and rapidly adapt defenses.
J. Detailed Analytics: Continuous monitoring provides detailed analytics on network traffic, system changes, and user behavior. These insights can be invaluable for identifying security holes and crafting effective defense strategies.
K. Network Visibility: Continuous monitoring offers a high level of network visibility. This visibility is crucial for understanding normal network behavior, which, in turn, helps in identifying anomalies indicative of a potential breach.
L. Automated Alerts: Automated alerts generated by continuous monitoring systems notify security teams about potential threats or unusual activities. This enables a proactive response and reduces the manual effort required for monitoring.
M. Protecting Sensitive Data: Continuous monitoring helps protect sensitive data by identifying and preventing unauthorized access or data exfiltration attempts. This is especially important for organizations handling confidential or personal information.
N. Behavioral Analytics: Leveraging behavioral analytics, continuous monitoring systems can establish a baseline of normal user behavior. Deviations from this baseline can be indicative of a security threat, prompting further investigation.
O. Threat Intelligence Integration: Continuous monitoring often integrates with threat intelligence feeds, allowing organizations to stay informed about the latest threats and vulnerabilities. This integration enhances the ability to detect and respond to emerging risks.
P. Cyber Resilience: By continuously monitoring and adapting to the evolving threat landscape, organizations build cyber resilience. This resilience is crucial for withstanding and recovering from cyberattacks and data breaches.
Q. Forensic Analysis: In the aftermath of a security incident, continuous monitoring facilitates detailed forensic analysis. It provides a comprehensive record of events, aiding in the investigation of the breach’s origin and impact.
R. Increased efficiency: Continuous monitoring can help organizations to improve the efficiency of their security operations by automating many of the tasks involved in threat detection and response.
iii. How to implement continuous monitoring
There are a number of steps that organizations can take to implement continuous monitoring, including:
A. Identify the data sources that will be monitored: Organizations should identify the data sources that contain the most valuable and sensitive data. This data should be prioritized for monitoring.
B. Choose the right monitoring tools: There are a number of different monitoring tools available, so it is important to choose the right tools for the organization’s needs.
C. Implement the monitoring tools: The monitoring tools should be implemented according to the organization’s policies and procedures.
D. Monitor the data: The data should be monitored on a regular basis to identify any anomalies or suspicious activity.
E. Respond to threats: Organizations should have a plan in place for responding to threats that are detected through continuous monitoring.
By following these tips, organizations can implement a successful continuous monitoring program that will help to protect their data from breaches.
Continuous monitoring is a vital tool for today’s organizations to protect their digital assets. By providing real-time visibility into their security posture, it empowers organizations to identify and respond to threats immediately, thereby mitigating risks of data breaches.
It truly acts as a shield, protecting businesses from the escalating threats that come with an increasingly digital world.
https://secureframe.com/blog/continuous-monitoring-cybersecurity