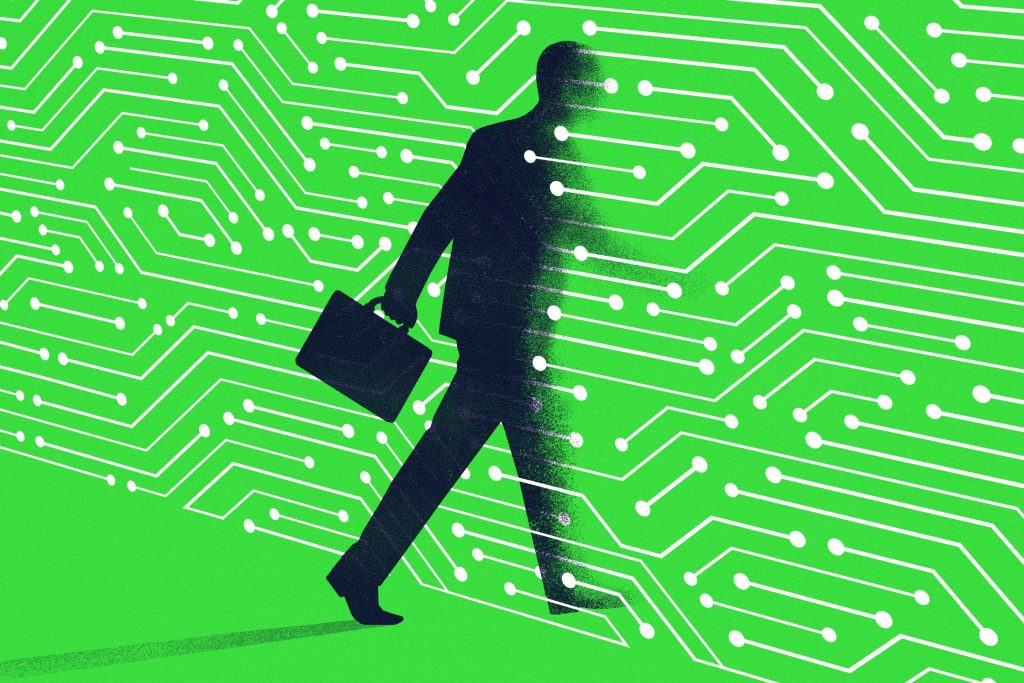
Artificial intelligence (AI) is revolutionizing industries, high cost hampers adoption
In the dynamic landscape of technological innovation, Artificial Intelligence (AI) stands as a beacon of promise, offering unparalleled opportunities for businesses to streamline operations, enhance productivity, and gain a competitive edge.
However, despite its transformative potential, the widespread adoption of AI among IT clients has been hindered by one significant barrier: the high cost associated with implementation.
The allure of AI is undeniable. From predictive analytics to natural language processing, AI-powered solutions offer businesses the ability to automate tasks, extract valuable insights from data, and deliver personalized experiences to customers. Yet, for many IT clients, the prospect of integrating AI into their operations is often accompanied by daunting price tags.
i. The Financial Barriers to AI Adoption
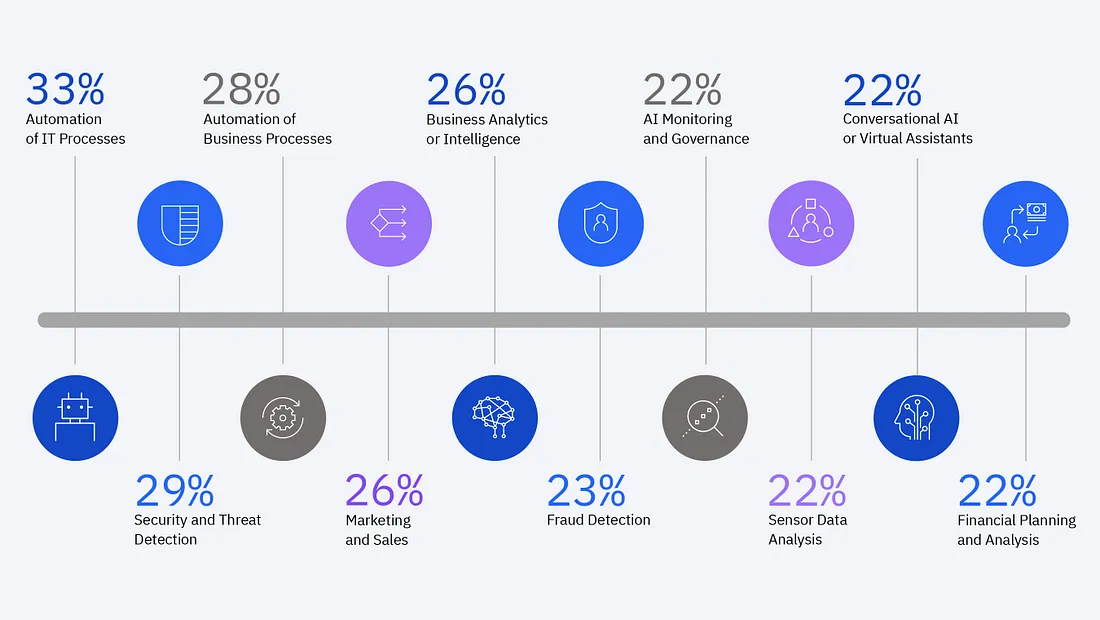
A. Initial Investment Costs
The initial investment required to integrate AI systems is substantial. For many businesses, particularly small and medium-sized enterprises (SMEs), the costs are daunting. AI implementation is not just about purchasing software; it also involves substantial expenditure on infrastructure, data acquisition, system integration, and workforce training. According to a survey by Deloitte, initial setup costs are among the top barriers to AI adoption, with many IT clients struggling to justify the high capital investment against uncertain returns.
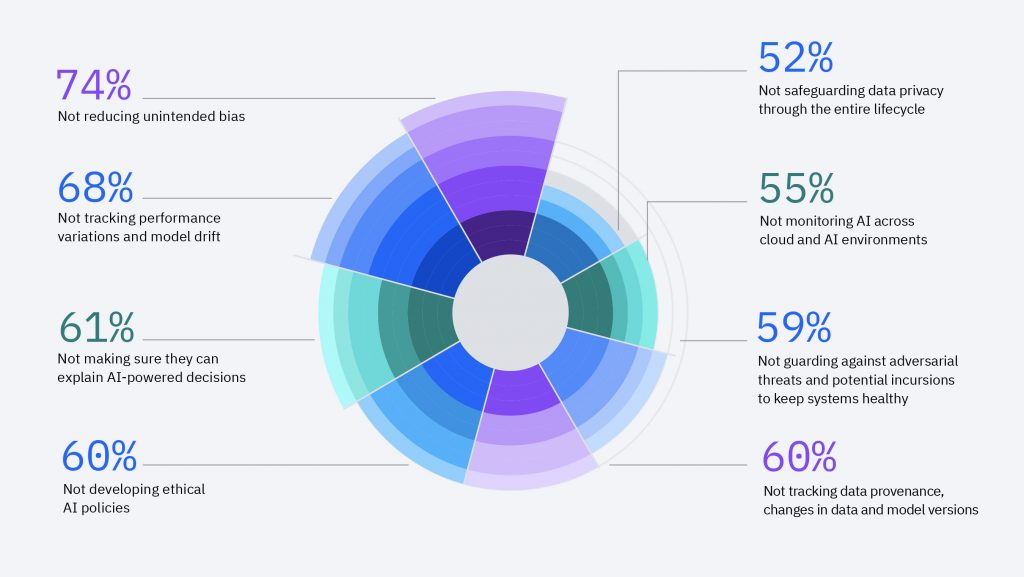
B. Operational Costs and Scalability Issues
Once an AI system is in place, operational costs continue to pile up. These include costs associated with data storage, computing power, and ongoing maintenance. Moreover, AI models require continuous updates and improvements to stay effective, adding to the total cost of operation. For many organizations, especially those without the requisite scale, these ongoing costs can prove unsustainable over time.
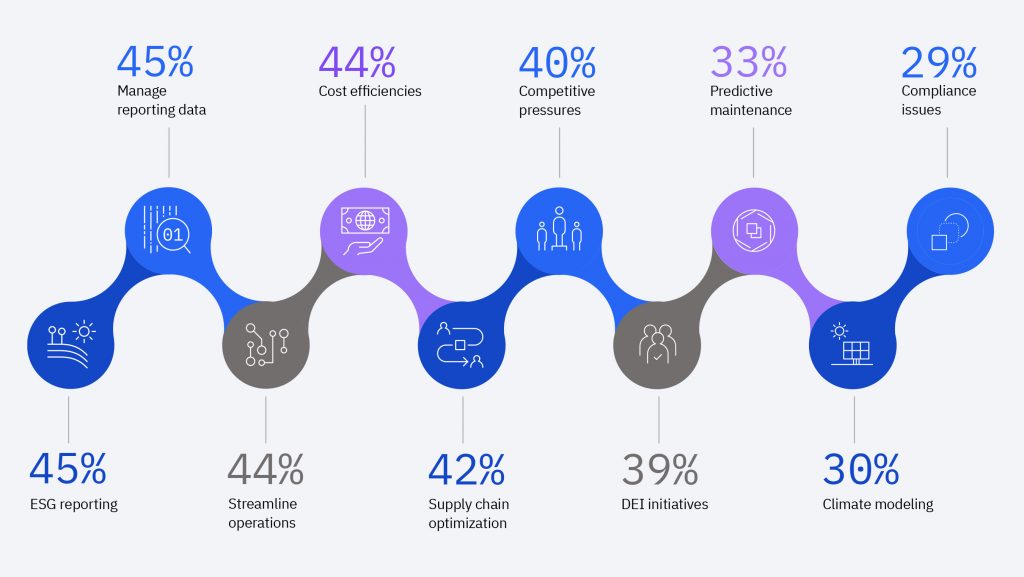
C. Skill Shortages and Training Expenses
Deploying AI effectively requires a workforce skilled in data science, machine learning, and related disciplines. However, there is a significant skill gap in the market, and training existing employees or hiring new specialists involves considerable investment in both time and money.
ii. Factors Compounding the Cost Issue
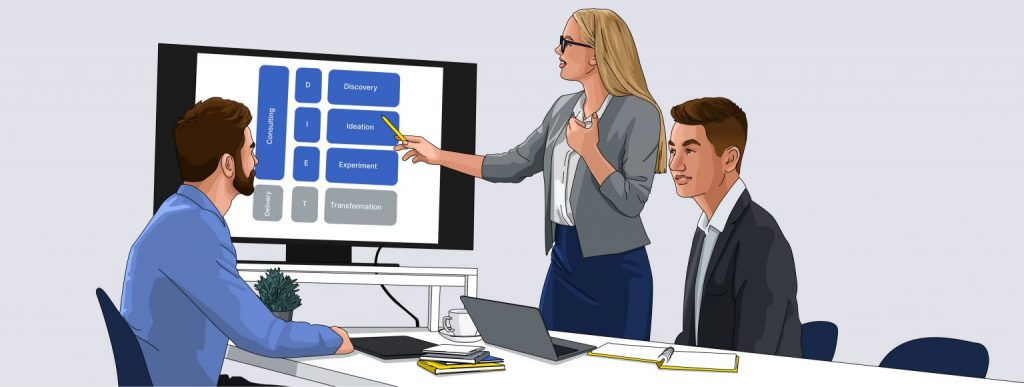
o Complexity and Customization: AI systems often need to be tailored to meet the specific needs of a business. This bespoke development can add layers of additional expense, as specialized solutions typically come at a premium.
o Data Management Needs: AI systems are heavily reliant on data, which necessitates robust data management systems. Ensuring data quality and the infrastructure for its management can further elevate costs, making AI adoption a less attractive prospect for cost-sensitive clients.
o Integration and Scalability Challenges: For AI systems to deliver value, they must be integrated seamlessly with existing IT infrastructure—a process that can reveal itself to be complex and costly. Moreover, scalability issues might arise as business needs grow, necessitating additional investment.
iii. Case Studies Highlighting Adoption Challenges
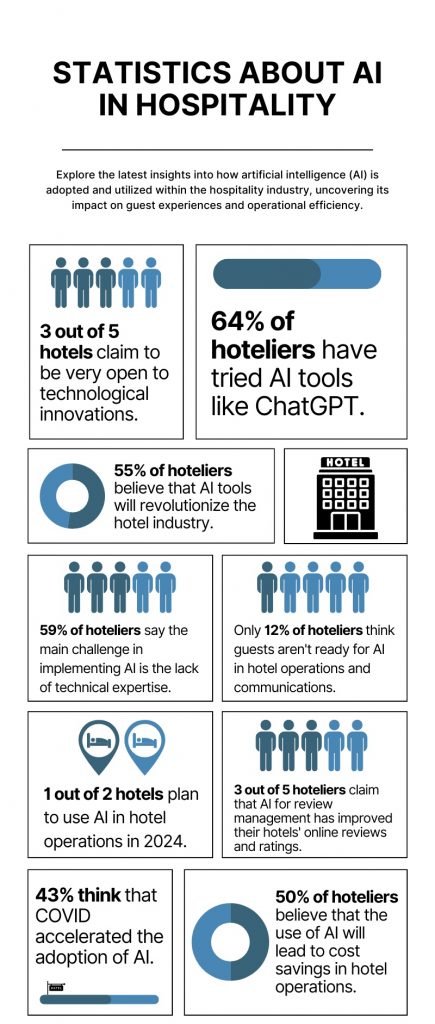
Several case studies illustrate how high costs impede AI adoption.
A. A mid-sized retail company attempted to implement an AI system to optimize its supply chain. The project required considerable upfront investment in data integration and predictive modeling. While the system showed potential, the company struggled with the ongoing costs of data management and model training, eventually leading the project to a standstill.
B. A healthcare provider looking to adopt AI for patient data analysis found the cost of compliance and data security to be prohibitively high. The additional need for continuous monitoring and upgrades made the project economically unfeasible in the current financial framework.
iv. The Broader Implications
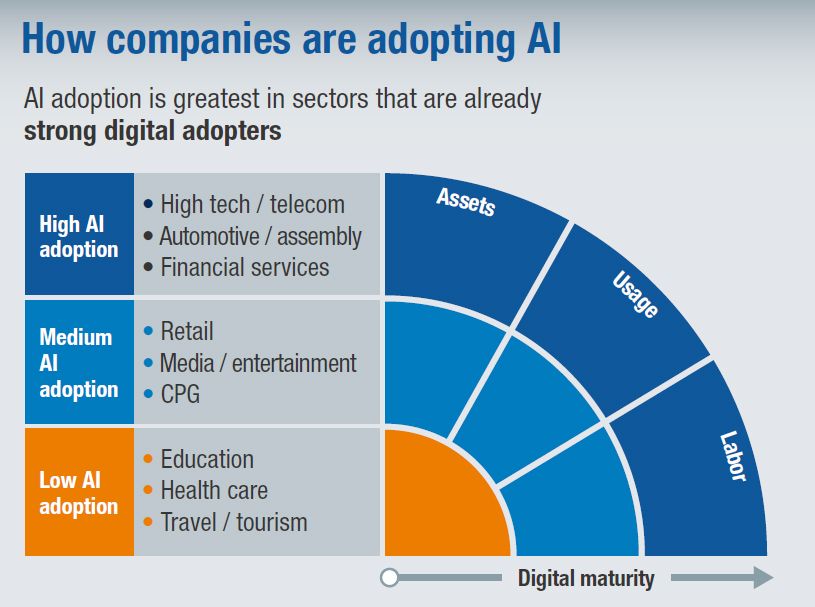
The high cost of AI adoption has significant implications for the competitive landscape. Larger corporations with deeper pockets are better positioned to benefit from AI, potentially increasing the disparity between them and smaller players who cannot afford such investments. This can lead to a widened technological gap, benefiting the few at the expense of the many and stifling innovation in sectors where AI could have had a substantial impact.
v. Potential Solutions and Future Outlook
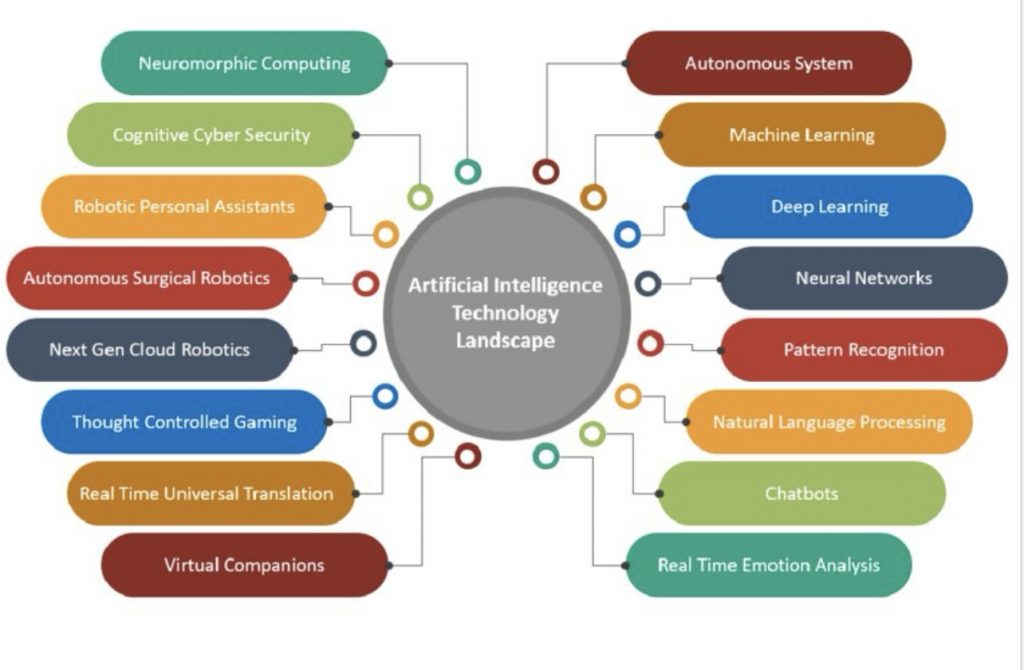
o Open Source and Cloud-Based AI Solutions: One potential way to mitigate high costs is through the use of open-source AI software and cloud-based AI services, which can offer smaller players access to sophisticated technology without requiring large upfront investments or in-house expertise.
o AI as a Service (AIaaS): Companies can also look towards AIaaS platforms which allow businesses to use AI functionalities on a subscription basis, reducing the need for heavy initial investments and long-term commitments.
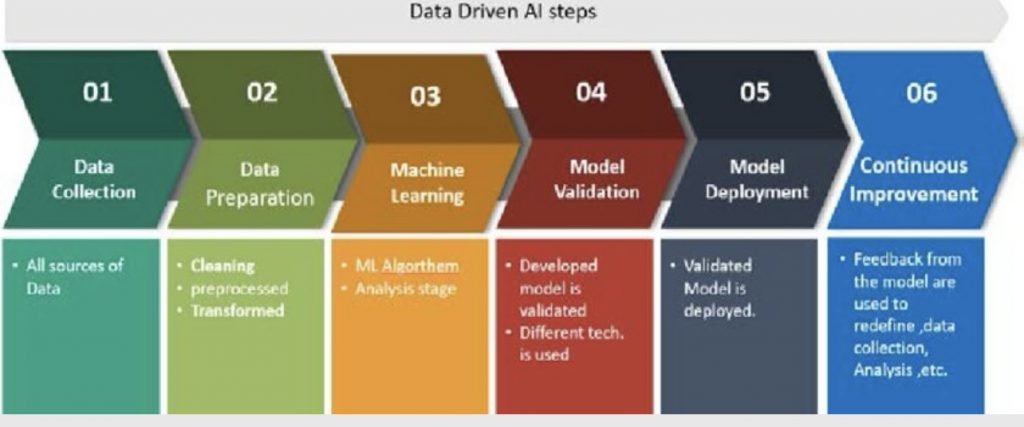
o Government and Industry-Led Initiatives: To support SMEs, governmental bodies and industry groups can offer funding, subsidies, training programs, and support to help democratize access to AI technologies.
o Partnerships between academic institutions and industry: Can facilitate the development of tailored AI solutions at a reduced cost, while simultaneously nurturing a new generation of AI talent.
vi. Conclusion
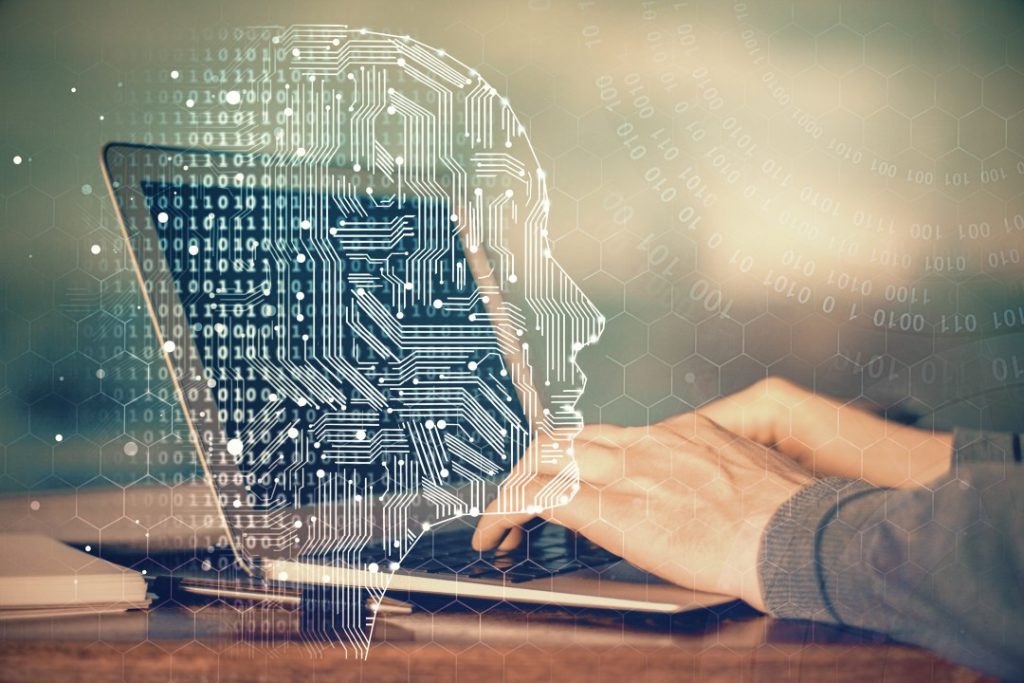
While AI technology holds transformative potential for businesses across sectors, the high cost associated with its adoption poses a formidable challenge.
For AI to reach its full potential and avoid becoming a tool only for the economically advantaged, innovative solutions to reduce costs and enhance accessibility are crucial.
By addressing these financial hurdles through innovative solutions and supportive policies, the path to AI integration can be smoothed for a wider range of businesses, potentially unleashing a new era of efficiency and innovation across industries.
Addressing these challenges will be key in ensuring that AI technologies can benefit a broader spectrum of businesses and contribute more evenly to economic growth. This requires concerted efforts from technology providers, businesses, and policymakers alike.
Yet, for now, the cost remains a pivotal sticking point, steering the discourse on AI adoption in the IT sector.
vii. Further references
Plain Conceptshttps://www.plainconcepts.com › a…Why AI adoption fails in business: Keys to avoid it
IBM Newsroomnewsroom.ibm.comData Suggests Growth in Enterprise Adoption of AI is Due to Widespread …
LinkedIn · Subrata Das10+ reactions · 4 years agoFactors inhibiting AI adoption
UiPathhttps://www.uipath.com › blog › ov…3 common barriers to AI adoption and how to overcome them
RT Insightshttps://www.rtinsights.com › ai-ad…AI Adoption is on the Rise, But Barriers Persist
CIO | The voice of IT leadershiphttps://www.cio.com › article › 9-…9 biggest hurdles to AI adoption
Exposithttps://www.exposit.com › BlogOvercoming Barriers to AI Adoption: A Roadmap …