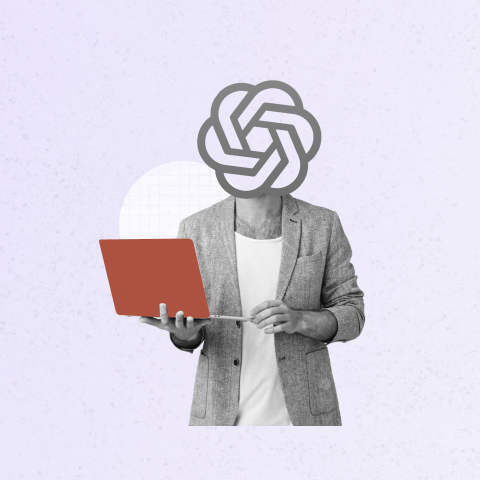
The Imperative Need for Internal Generative AI Policies
In an era where technological advancements are continuous and rapid, generative Artificial Intelligence (AI) stands out as a particularly transformative technology.
Generative AI refers to the class of AI that can generate text, images, sounds, and other types of media, simulating human-like creativity.
However, with great power comes great responsibility, and as AI capabilities expand, so too do the ethical considerations surrounding its use. One crucial aspect that demands attention is the establishment of internal generative AI policies within organizations.
i. The Power of Generative AI
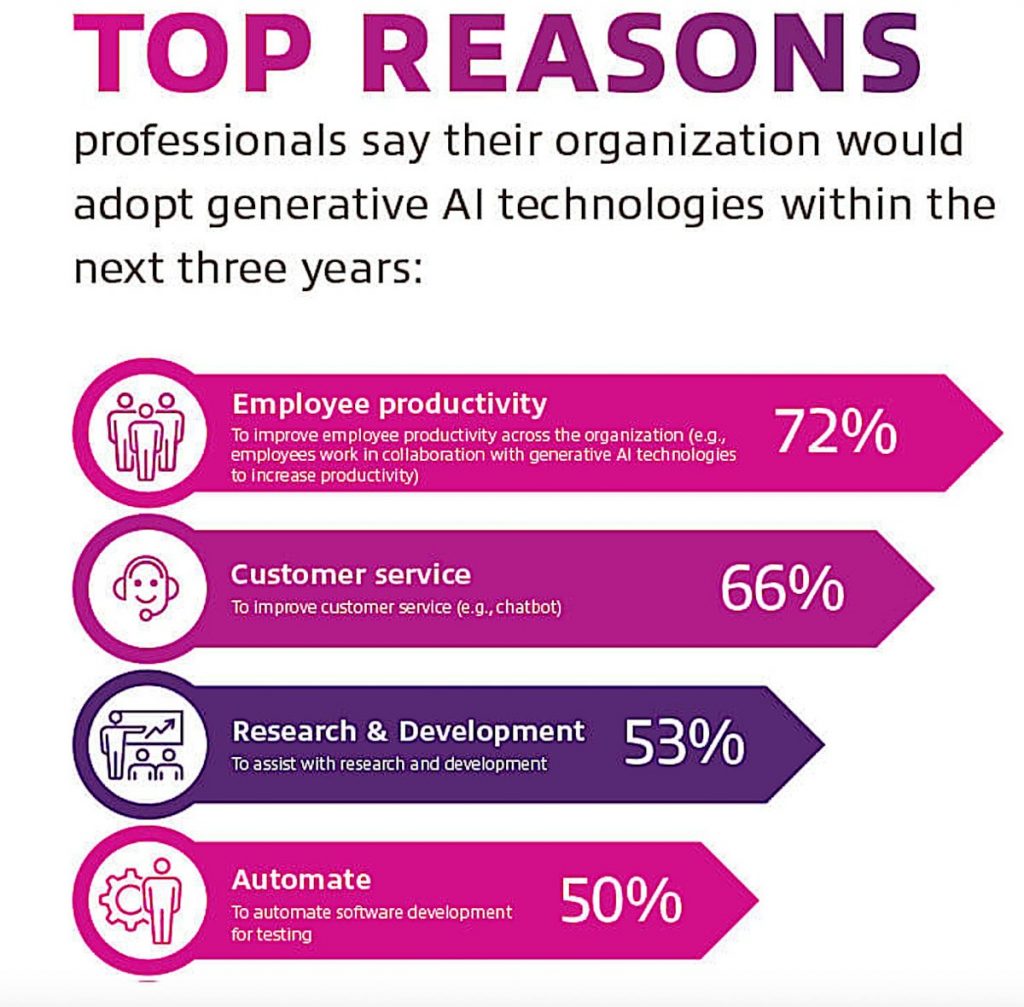
GenAI excels at creating entirely new content from data. This translates to a multitude of benefits:
o Enhanced Creativity and Innovation: GenAI can generate fresh ideas for marketing campaigns, product designs, or even scientific research.
o Content Creation at Scale: Automate content generation for social media, marketing materials, or internal reports, freeing up human resources.
o Improved Efficiency: Streamline workflows and accelerate processes by leveraging AI-generated data and content.
ii. Some perils of Generative AI
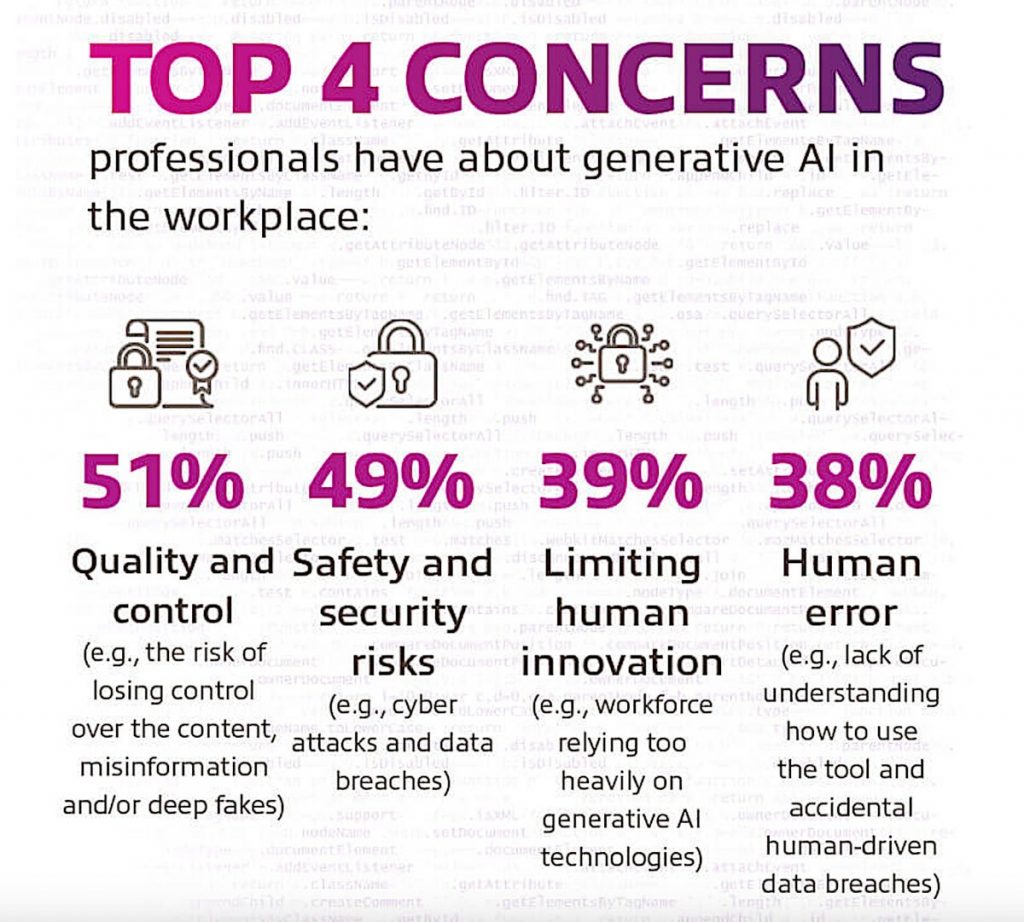
o Bias and Misinformation: GenAI models can perpetuate biases present in their training data. This can lead to discriminatory or misleading content generation.
o Security and Privacy Concerns: The vast amounts of data used to train GenAI models raise security and privacy issues that require careful consideration.
o Over-reliance and Lack of Transparency: Overdependence on GenAI-generated content without human oversight can lead to a lack of transparency and authenticity.
o Intellectual Property and Copyright Concerns: Generative AI can easily produce materials that might infringe on copyrights, intentionally or unintentionally.
iii. Internal Policies: Safeguarding the Future
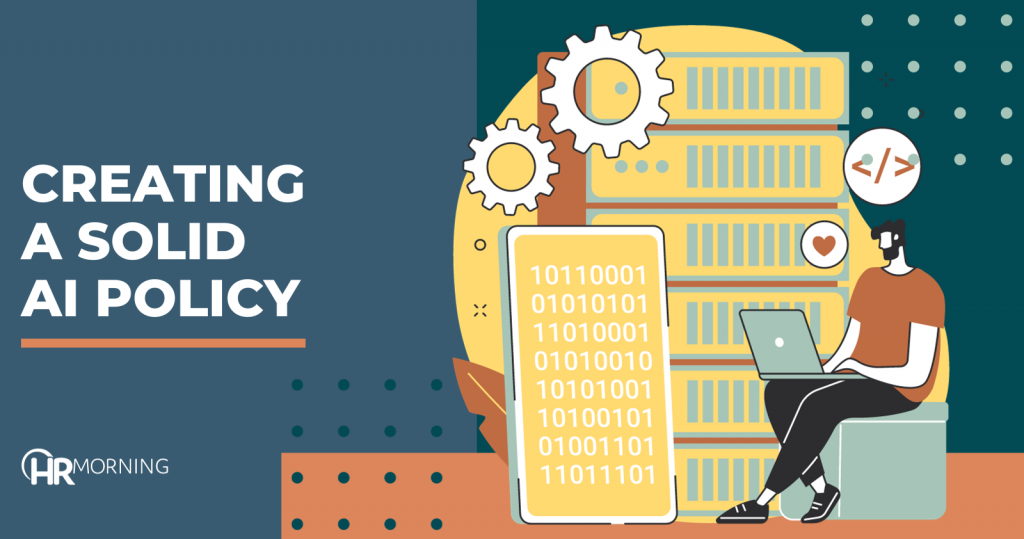
To harness the power of GenAI responsibly, businesses need internal policies that address these concerns.
Here’s what the policy should cover:
A. Ensuring Ethical Use
The ability of generative AI to create convincing content that can mimic human output raises immediate ethical questions. For instance, AI can be used to generate misleading information or deepfake content that can misinform or harm individuals or groups. Internal policies must address these issues directly, ensuring that the use of generative AI aligns with ethical standards that uphold truth and integrity while prohibiting uses that can deceive or harm.
B. Protecting Intellectual Property
Generative AI systems often learn from large datasets that may include copyrighted materials. Organizations need to establish clear guidelines on the training processes for AI to avoid legal repercussions related to copyright infringement. Furthermore, there is also the necessity to address the ownership of AI-generated content, determining whether it belongs to the AI developers, the organization, or is considered a derivative work.
C. Ensuring Data Privacy
AI systems, particularly those that generate predictive text or content, rely heavily on data which might include sensitive or personal information. Organizations must draft policies that conform with global data protection regulations like GDPR in Europe or CCPA in California, ensuring data privacy and security are upheld, thereby maintaining the trust of users and stakeholders.
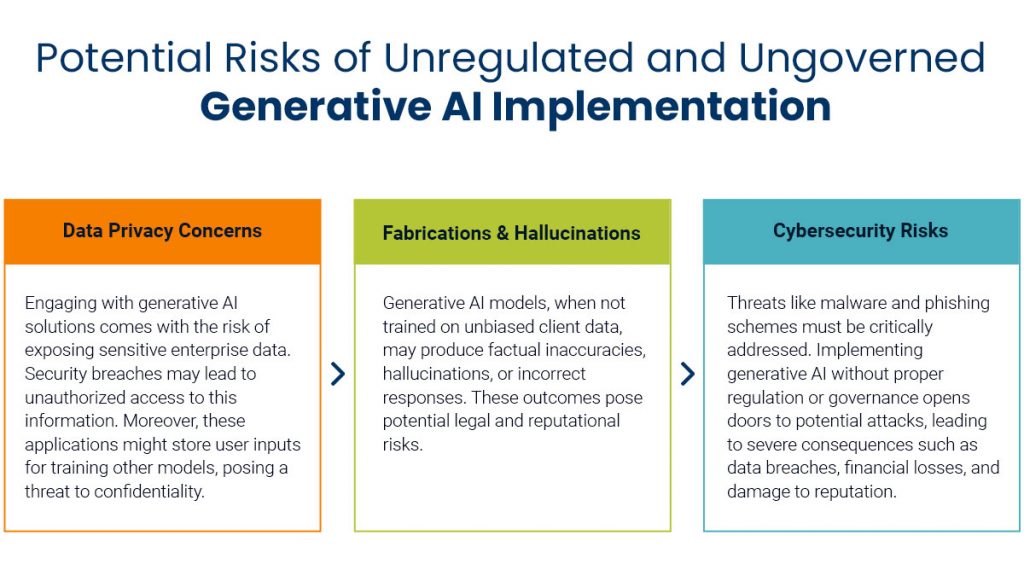
D. Governance and Oversight
The deployment of generative AI should be monitored and managed to prevent unintended consequences. Effective governance policies should include oversight mechanisms that regularly evaluate the AI’s output and its alignment with organizational goals and ethical standards. This oversight should also extend to continuously assessing the AI’s decision-making patterns to ensure they do not develop or exhibit biased behaviors.
E. Transparency and Accountability
It is crucial for organizations to be transparent about how they utilize generative AI technologies. Stakeholders, including customers and the general public, have the right to know how their data might be used or how decisions are being influenced by AI. Policies should ensure transparency in AI operations and clearly define accountability in cases of failure or when unintended harm is caused.
F. Employee Training and Awareness
With generative AI often embedded in various functions across an organization, employees must understand the technology’s capabilities and limitations. Internal policies should include comprehensive training programs that educate employees about both operational and ethical aspects of using generative AI. This education can foster a knowledgeable workforce that can leverage AI responsibly.
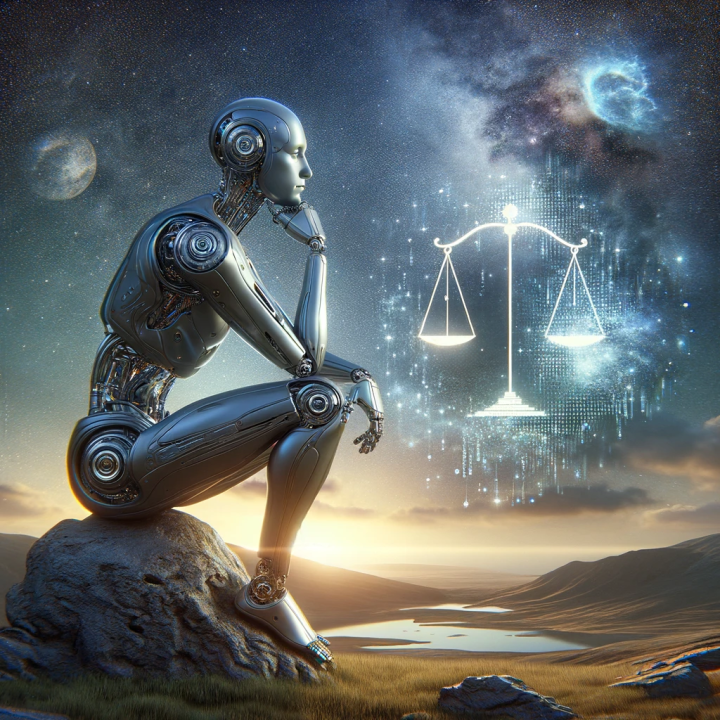
G. Legal Compliance
With the proliferation of data protection regulations like GDPR and CCPA, organizations must ensure that their AI initiatives comply with relevant laws and regulations. Internal generative AI policies provide clarity on legal requirements, data privacy measures, and accountability mechanisms, reducing the risk of regulatory violations and associated penalties. Internal policies should detail procedures for regular legal audits and updates in accordance with changing legislation.
H. Risk Management
AI systems are not immune to vulnerabilities and risks. From cybersecurity threats to algorithmic errors, the deployment of AI carries inherent risks that must be mitigated. Internal generative AI policies incorporate risk assessment protocols, cybersecurity measures, and contingency plans to minimize potential harms and safeguard organizational interests.
I. Alignment with Organizational Values
Every organization operates within a unique cultural and ethical context. Internal generative AI policies reflect the values and principles that define an organization’s identity. By aligning AI initiatives with these core values, organizations can ensure that their technological advancements contribute positively to society while upholding integrity and respect for human rights.
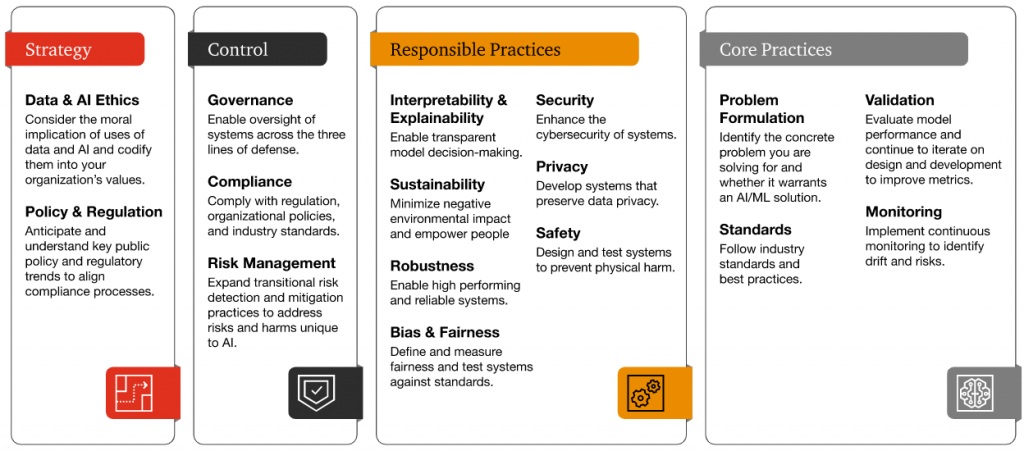
J. Continuous Improvement
AI technology is constantly evolving, and so too should the policies that govern its use. Internal generative AI policies are dynamic frameworks that adapt to changing technological landscapes, emerging ethical dilemmas, and stakeholder feedback. By fostering a culture of continuous improvement, organizations can stay ahead of the curve and maintain ethical leadership in the AI space.
iv. Steps to Establish Effective Internal Generative AI Policies
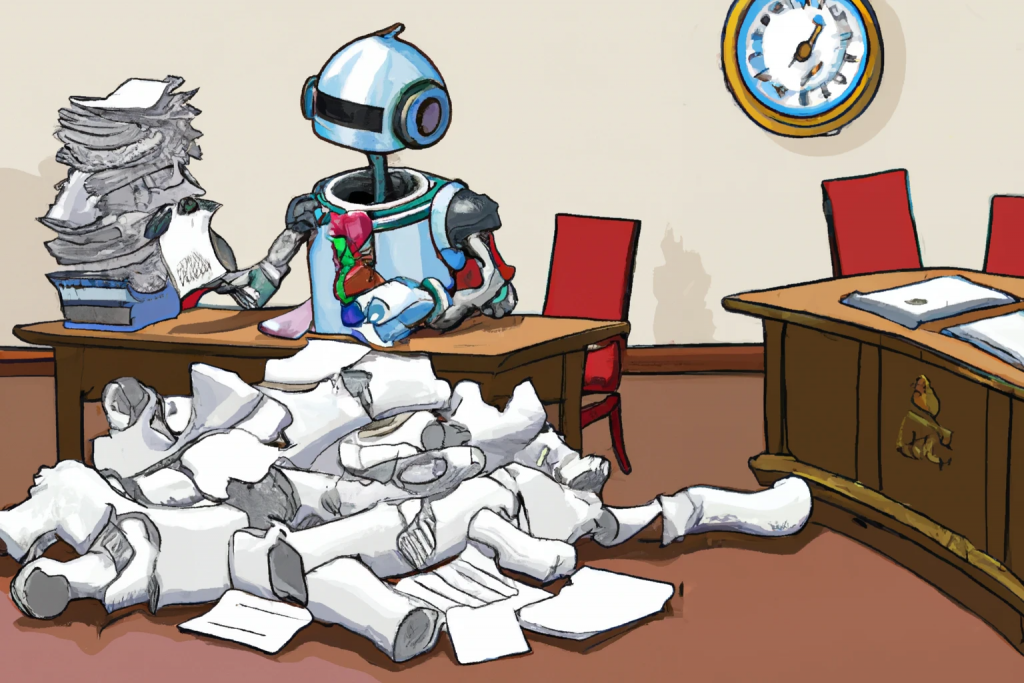
A. Assess AI Readiness
Organizations should begin by assessing their current technology landscapes and defining the scope of AI integration in line with their strategic goals. This involves understanding the capabilities of AI and the areas where it can add the most value.
B. Draft Clear Usage Guidelines
Based on the readiness assessment, clear guidelines regarding the appropriate uses of generative AI within the organization should be established. This includes defining permissible and non-permissible actions for AI systems.
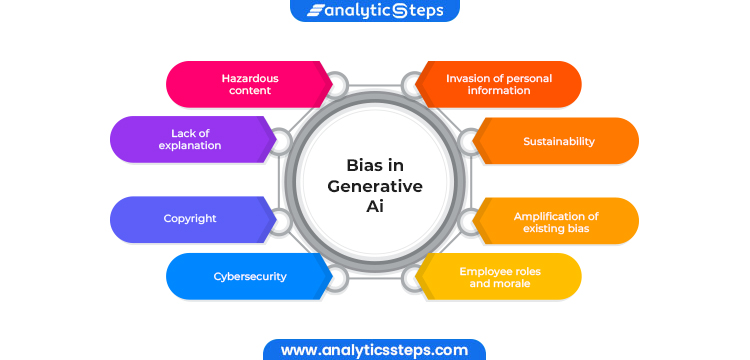
C. Implement Training Programs
Comprehensive training programs for employees to understand and effectively use generative AI are crucial. Such training helps mitigate fear and misunderstanding about AI while promoting innovative uses of AI tools within the firm.
E. Regularly Review and Update Policies
AI and related technologies are continuously evolving. Regularly reviewing and updating AI policies ensure that organizations remain compliant with new regulations and technological advancements.
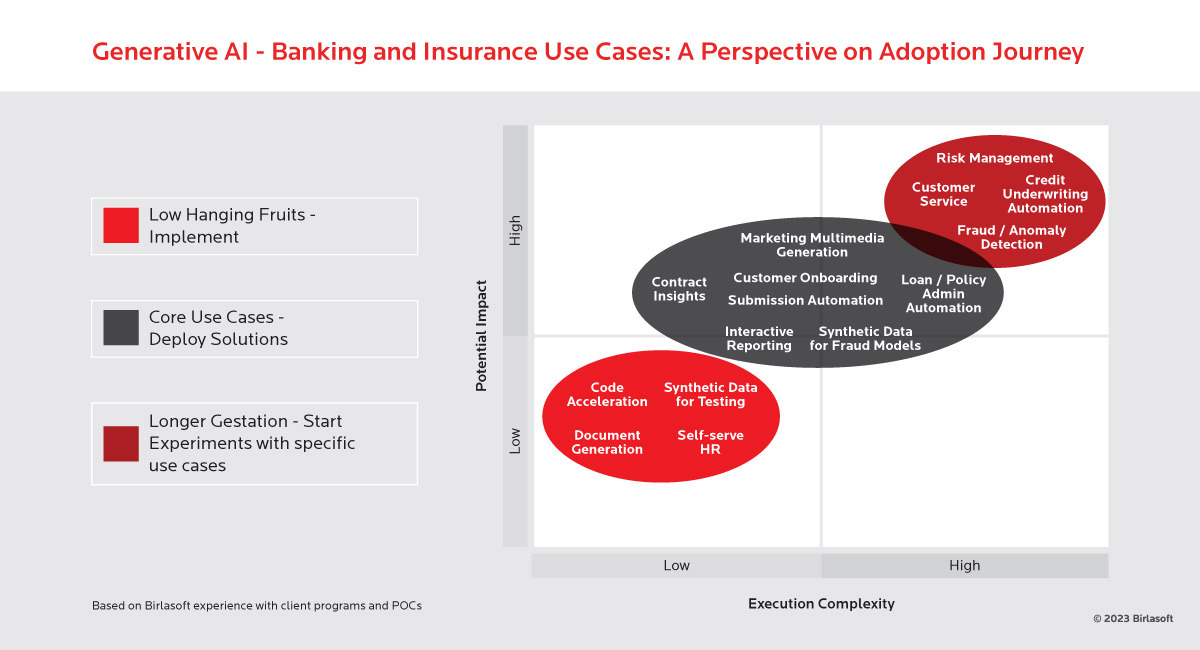
F. Foster a Culture of Responsible AI Use
Creating a culture that prioritizes ethical AI use goes a long way in ensuring compliance and innovation. This involves leadership endorsement, clear communication about the benefits and challenges of AI, and a commitment to ethical practices as a core organizational value.
v. The Generative AI Advantage
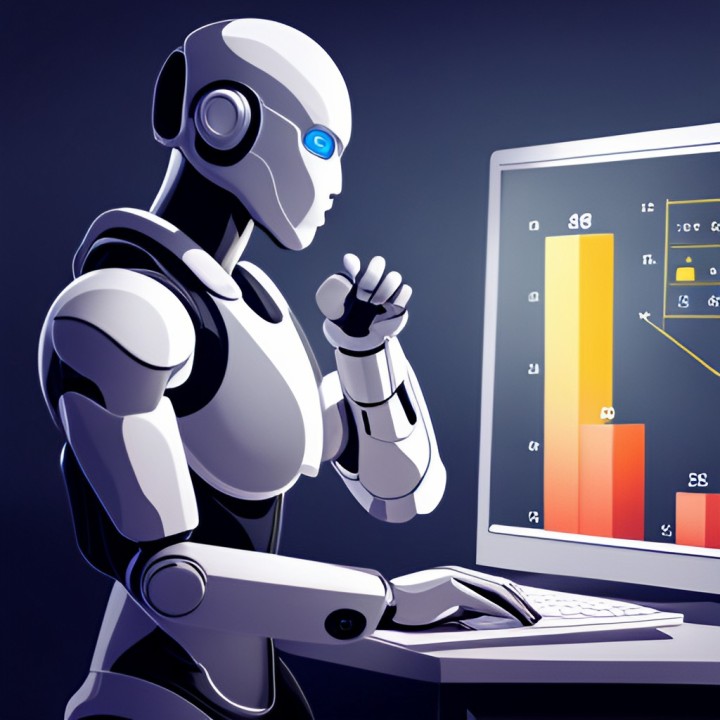
By implementing a well-defined internal generative AI policy, businesses can:
o Minimize Risks: Proactive measures mitigate the potential downsides of GenAI, safeguarding your brand reputation and user trust.
o Foster Innovation: Clear guidelines empower employees to leverage GenAI responsibly, fostering a culture of innovation within a controlled framework.
o Maintain Ethical Use: A strong policy ensures your organization sets a positive example for ethical and responsible GenAI integration.
vi. Conclusion
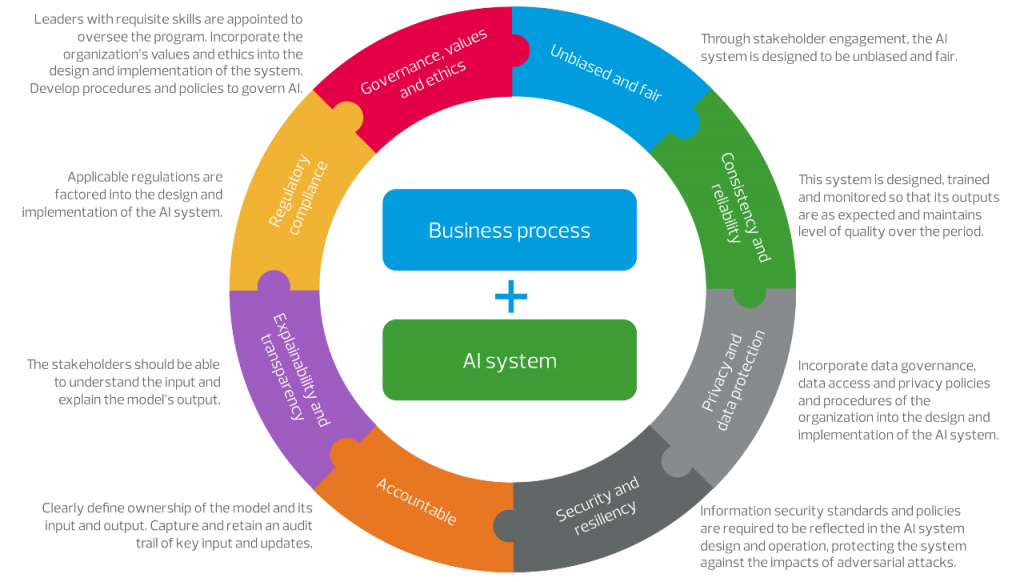
In conclusion, the adoption of internal generative AI policies is not just a matter of compliance or risk management; it is a strategic imperative for organizations committed to ethical innovation.
By establishing clear guidelines, promoting transparency, and upholding core values, these policies empower organizations to harness the potential of AI while mitigating risks and safeguarding societal well-being.
As AI continues to shape our world, proactive governance through internal generative AI policies will be instrumental in ensuring that its impact remains positive and beneficial for all.
vii. Further references
HRMorninghttps://www.hrmorning.com › gen…Generative AI policy for the workplace: 5 keys to include
Skillsofthttps://www.skillsoft.com › blogHow to Write an AI Policy for Your Organization