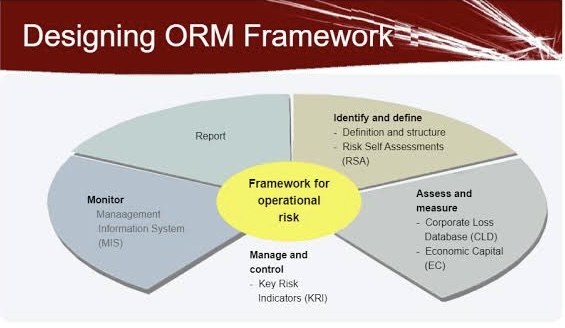
A Loss Data Program accounts for historical loss data that can be instrumental in an Operational Risk Management Framework.
This program can help organizations identify, track, and analyze past operational risk events, providing valuable insight into potential vulnerabilities and potential areas for improvement.
i. Key Purpose:
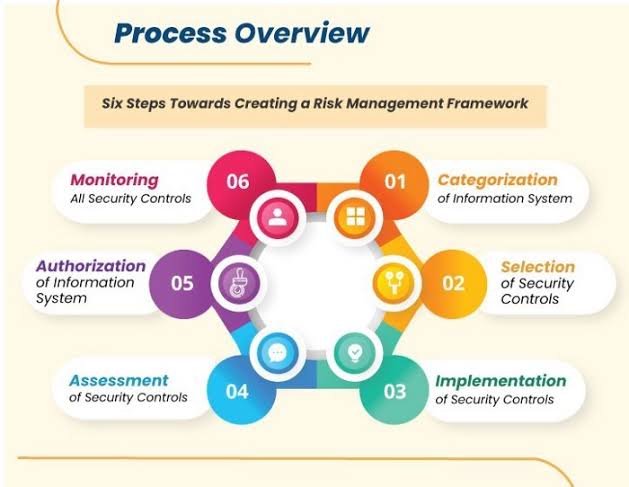
o To collect, store, analyze, and report operational loss events to:
o Identify and understand risk patterns
o Quantify potential losses
o Improve risk management decisions
o Allocate capital reserves appropriately
ii. Key Components:
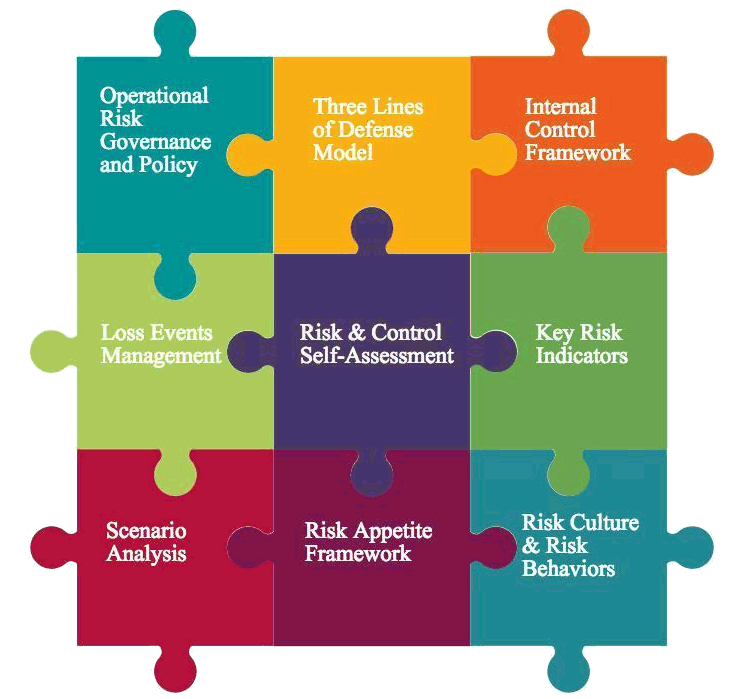
A. Loss Data Collection:
o Scope: Defining which events to capture (internal, external, near misses, etc.)
o Thresholds: Setting minimum financial loss amounts for inclusion
o Data Fields: Establishing standard fields for recording details (e.g., date, business line, cause, loss amount)
o Data Sources: Identifying and accessing relevant data sources (e.g., incident reports, financial systems, insurance claims)
B. Loss Data Storage:
o Database: Selecting a secure and accessible database for storage
o Data Quality: Ensuring accuracy, completeness, and consistency of data
o Data Governance: Establishing policies for data access, usage, and retention
C. Loss Data Analysis:
o Categorization: Classifying losses by event type, business line, cause, etc.
o Frequency and Severity Analysis: Assessing the frequency and magnitude of losses
o Trend Analysis: Identifying patterns and trends over time
o Root Cause Analysis: Investigating underlying causes of losses
o Scenario Analysis: Modeling potential future losses
D. Loss Data Reporting:
o Regular Reports: Generating reports for management, board, and regulators
o Key Risk Indicators (KRIs): Tracking metrics to monitor risk levels
iii. Benefits of a Robust Loss Data Program:
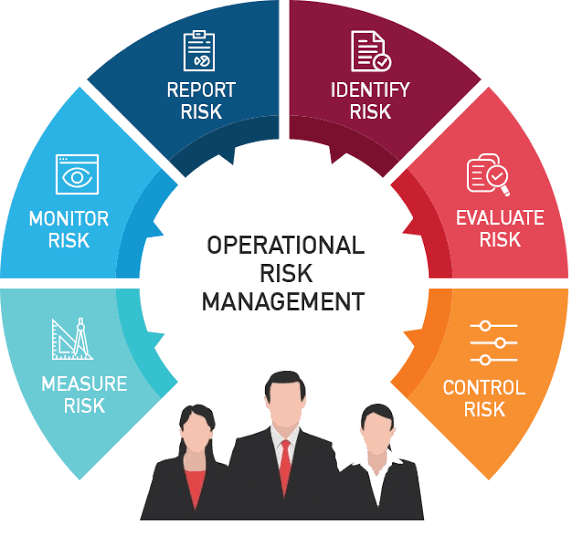
o Enhanced risk awareness and understanding
o Improved decision-making for risk mitigation and control
o More accurate capital allocation
o Proactive identification of emerging risks
o Strengthened compliance with regulatory requirements
iv. Key steps to integrating a Loss Data Program in an Operational Risk Management framework:
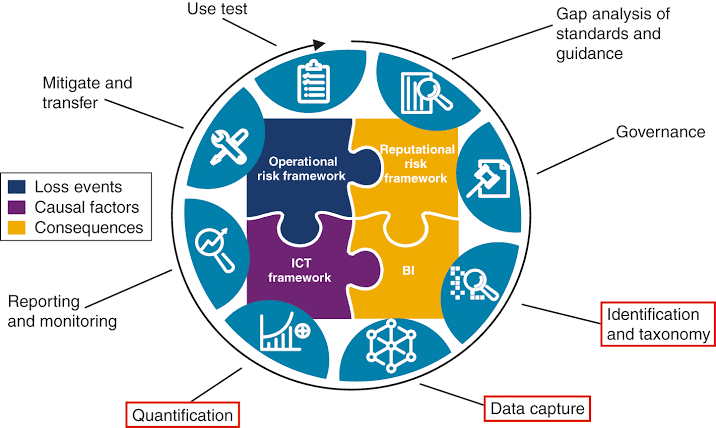
A. Data capture: Organizations need an efficient and consistent methodology for capturing data about operational risk loss events. This process involves identifying incidents, recording relevant information (such as the type of event, outcomes, causes, and loss amounts), and maintaining a database or system for storing this information.
B. Data Collection and Categorization:
o Establish a structured system for collecting data on operational losses. This includes incidents, near-misses, and actual losses.
o Categorize losses based on predefined risk categories, such as technology failures, human errors, external events, or process deficiencies.
C. Centralized Database:
o Maintain a centralized and accessible database to store loss data. This facilitates consistent data entry, retrieval, and analysis across the organization.
o Ensure data integrity and accuracy through regular reviews and validations.
D. Data classification: Once data is captured, it should be correctly classified according to event types related to operational risk, such as internal fraud, external fraud, employment practices and safety, clients, products & business practices, execution, delivery, and process management, among others.
E. Data Analysis: Analyze the data to discern patterns, trends, and areas of vulnerability. This may include assessing frequencies, identifying root causes, determining severity based on financial impact, and mapping losses to specific business lines and processes.
F. Loss Event Taxonomy:
o Establish a standardized taxonomy for loss events, ensuring consistency in reporting and analysis.
o This taxonomy aids in classifying events based on their characteristics and impacts, fostering a comprehensive understanding of the risk landscape.
G. Modeling and Scenario Analysis: Apply statistical techniques and risk models to the historical loss data to estimate potential losses. Also, use scenario analysis to explore outcomes from rare but plausible high-impact events. The choice of model will depend on the nature of operational risks the organization is exposed to and the type of data available.
H. Inform Risk Mitigation: Use the results of your analysis to inform risk mitigation strategies. This can include updating processes, implementing additional controls, refining early warning indicators, or purchasing insurance.
I. Key Risk Indicators (KRIs):
o Develop Key Risk Indicators based on historical loss data to provide early warnings of potential risk events.
o Align KRIs with strategic business objectives to ensure they are relevant and actionable.
J. Risk Appetite and Tolerance:
o Define risk appetite and tolerance levels based on the analysis of historical loss data. This assists in setting thresholds for acceptable levels of risk exposure.
o Align risk appetite with the organization’s strategic goals and objectives.
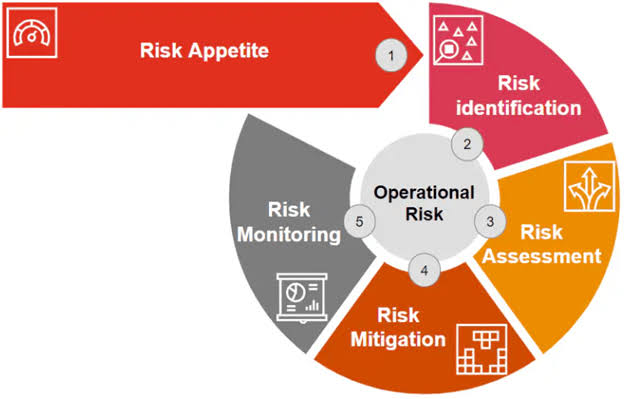
K. Thresholds for Reporting: Define the thresholds (e.g., monetary values or impact levels) that trigger mandatory reporting of a loss event within the organization.
L. Reporting: Regularly report the findings of the Loss Data Program to decision-makers. This should include clear explanations of the patterns or trends identified, their potential impacts, and any recommendations for risk mitigation.
M. Continuous Improvement:
o Foster a culture of continuous improvement by learning from past losses. Encourage feedback loops to ensure that lessons learned are applied to enhance risk controls and prevent recurrence.
o Periodically review and update the Loss Data Program to adapt to evolving business processes and emerging risks.
N. Regulatory Compliance:
o Ensure that the Loss Data Program aligns with regulatory requirements and industry standards.
o Regularly assess and update the program to incorporate changes in regulations that may impact operational risk management.
O. Communication and Training:
o Communicate the findings and insights derived from loss data analysis to relevant stakeholders.
o Provide training programs to enhance risk awareness and ensure that employees understand their roles in preventing and mitigating operational risks.
v. Utilizing the Loss Data:
A. Trends and Pattern Analysis: Regularly review the data to identify patterns or trends in loss events, which can help pinpoint systemic issues or areas of vulnerability.
B. Risk Assessment and Modeling: Use historical loss data to quantify exposure to operational risks. This data may feed into statistical models or actuarial analysis to estimate potential losses and inform the risk appetite.
C. Control Effectiveness: Assess the effectiveness of current controls based on the frequency and severity of loss events. Where controls are failing, enhancements can be made.
D. Capital Allocation: Inform the capital allocation process by estimating the potential impact of operational losses. Firms may set aside capital commensurate with their risk profile.
E. Performance Metrics: Develop metrics and indicators based on loss data for accountability and to monitor the performance of risk management activities.
F. Feedback Loop: Create a feedback loop where loss data informs risk management practices, training, and awareness programs, leading to continuous improvement of the operational risk framework.
G. External Sharing and Benchmarks: Where applicable, participate in industry loss data consortiums for benchmarking and gaining insights from the loss experiences of peers.
H. Regulatory Compliance and Reporting: Use collected data to fulfill regulatory reporting requirements regarding operational losses and risk management effectiveness.
Integrating a Loss Data Program into the Operational Risk Management Framework establishes a systematic and data-driven approach to identifying, assessing, and managing operational risks.
By leveraging historical loss data, organizations can strengthen their risk resilience, optimize risk mitigation efforts, and proactively address emerging threats within the dynamic business environment.
https://www.bis.org/bcbs/events/wkshop0303/p04deforose.pdf
https://www.bis.org/publ/bcbs195.pdf
https://www.auditboard.com/blog/operational-risk-management/