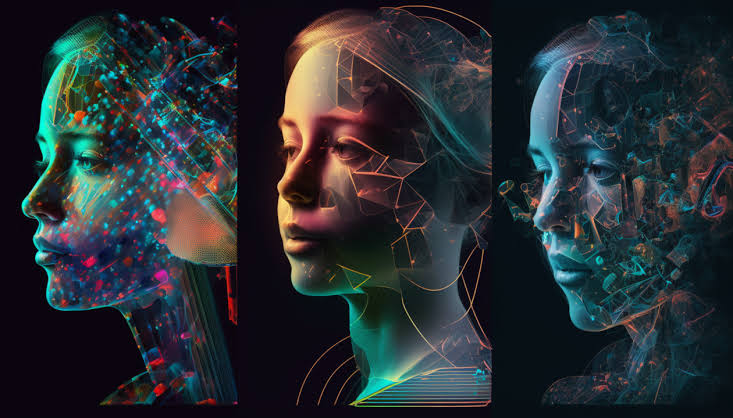
Generative AI and Cybersecurity: Impacting Defenses and Threats
In the rapidly evolving landscape of cybersecurity, the emergence of generative artificial intelligence (AI) heralds a new era of both opportunities and challenges. Generative AI, with its ability to create content and simulate human behavior, is at the forefront of transforming cybersecurity practices.
However, while it strengthens defenses by enhancing threat detection and response capabilities, it also amplifies potential threats by enabling more sophisticated cyberattacks.
i. Generative AI: A Primer
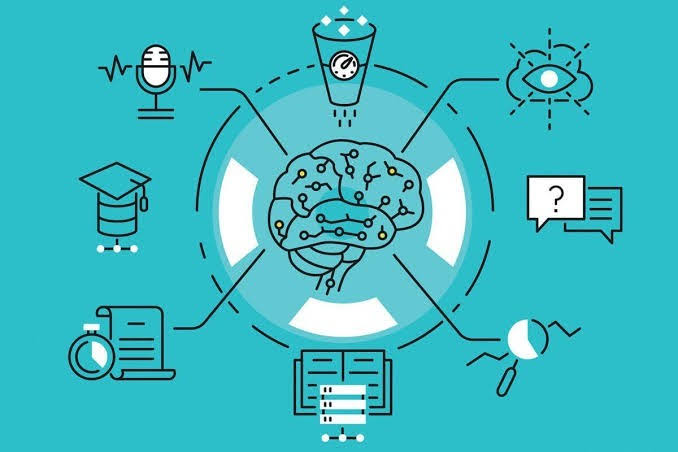
Generative AI refers to algorithms capable of creating content—ranging from text, images, and videos to code—after learning from extensive datasets. Unlike conventional AI models that are designed for recognition and classification tasks, generative AI can produce new, previously unseen outputs, making it a powerful tool for innovation. However, this capability also presents new challenges and opportunities within cybersecurity.
ii. Reinforcing Cyber Defenses with Generative AI
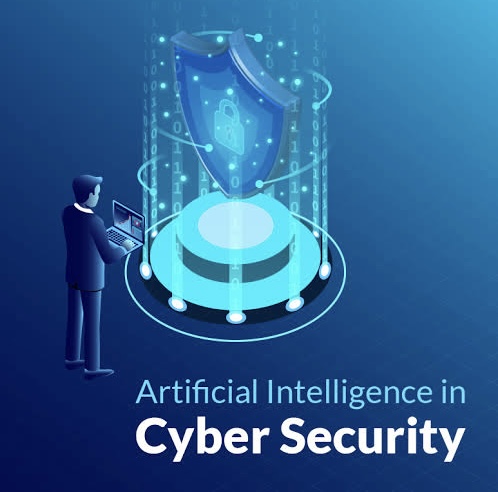
Generative AI introduces innovative ways to bolster cybersecurity defenses, primarily through advanced threat detection, dynamic security protocols, and improved cybersecurity training.
A. Threat Detection and Anomaly Recognition
Generative AI excels in analyzing vast datasets to identify patterns and anomalies that might signal a cyber threat. Machine learning algorithms, powered by generative models, can swiftly recognize deviations from normal behavior, enabling proactive threat detection. This capability significantly bolsters traditional cybersecurity measures, offering a dynamic defense mechanism against evolving threats.
B. Create security tools
AI can be used to develop new security software, such as firewalls and intrusion detection systems, that can adapt to evolving threats.
C. Predictive Analytics for Vulnerability Assessment
By harnessing generative AI, organizations can conduct advanced predictive analytics to identify potential vulnerabilities in their systems. These models simulate various attack scenarios, allowing cybersecurity professionals to preemptively address weak points in their infrastructure before they can be exploited by malicious actors.
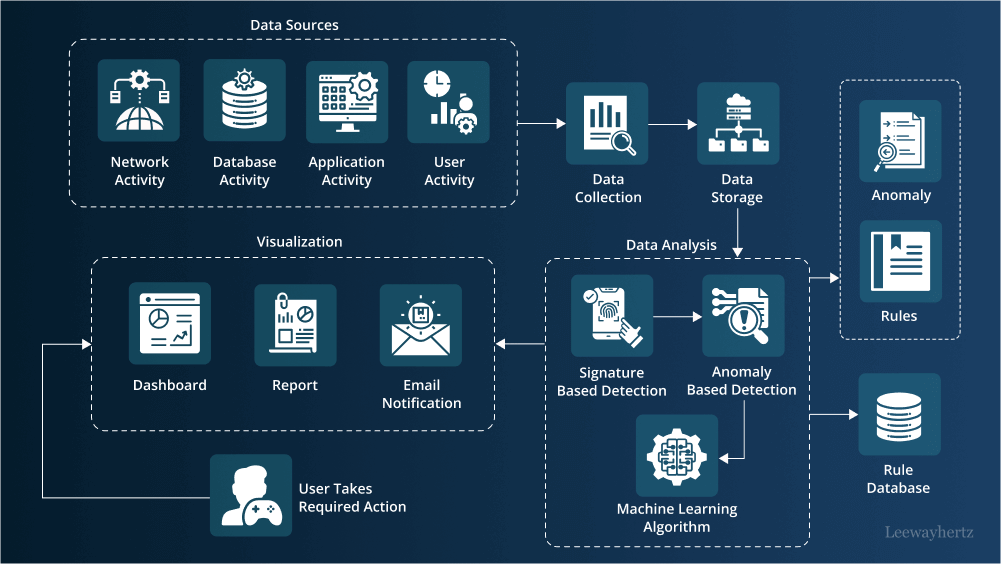
D. Automated Response and Mitigation
Generative AI facilitates the development of automated response systems that can counteract cyber threats in real-time. Through intelligent decision-making processes, these systems can isolate compromised components, patch vulnerabilities, and mitigate the impact of attacks swiftly, reducing the window of opportunity for adversaries.
E. Dynamic Security Protocols
Generative AI can assist in creating dynamic security protocols that adapt to the changing cyber threat landscape. By continuously learning from ongoing cyber activities, AI systems can recommend adjustments to security measures, ensuring they remain effective against evolving threats. This adaptability extends to the generation of complex, changing passwords and encryption keys, making unauthorized access increasingly difficult.
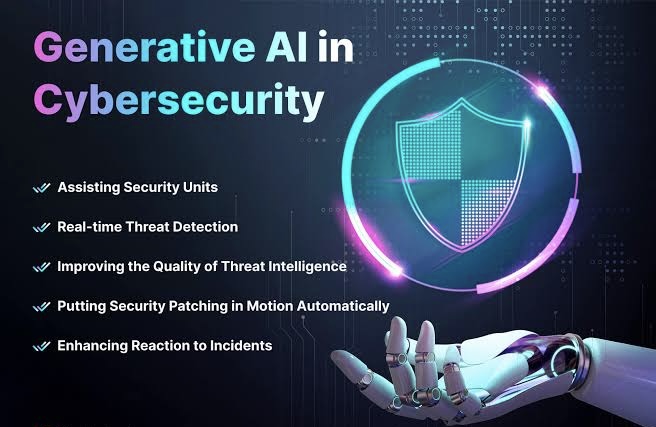
F. Improved Cybersecurity Training
Utilizing generative AI, organizations can develop realistic cyberattack simulations for training purposes, enhancing the preparedness of their cybersecurity teams. These simulations can replicate the tactics, techniques, and procedures (TTPs) of actual adversaries, offering a practical, hands-on experience that theoretical training methods cannot match.
iii. The Dark Side: Generative AI in the Hands of Adversaries
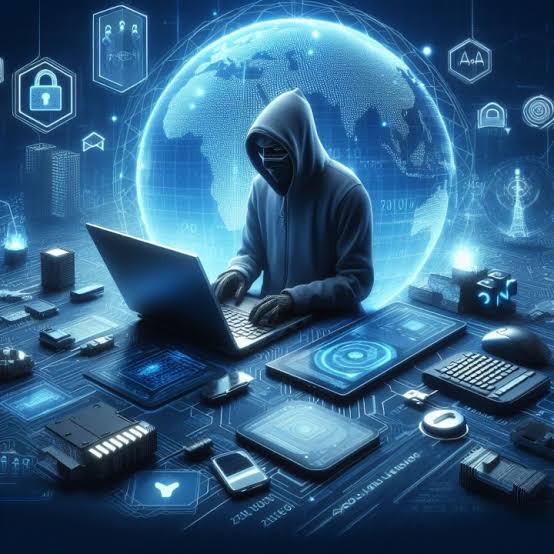
Conversely, the capabilities of generative AI that fortify cybersecurity defenses can also be exploited to conduct more advanced cyberattacks, raising significant concerns for cybersecurity professionals.
A. Sophisticated Phishing Attacks
Generative AI can craft highly convincing phishing emails and messages by analyzing communication patterns from social media and other sources. Such AI-generated messages can mimic the style and tone of genuine communications, increasing the likelihood of deceiving recipients into divulging sensitive information or downloading malicious software.
B. AI-Enhanced Social Engineering Attacks
Malicious actors are leveraging generative AI to refine social engineering attacks. Chatbots powered by AI can mimic human interactions convincingly, tricking users into divulging sensitive information. This sophisticated approach poses a significant challenge for traditional cybersecurity measures that often struggle to differentiate between genuine and AI-generated communication.
C. Evolving Malware and Adversarial Machine Learning
Generative AI is empowering the creation of adaptive malware that can evolve to evade traditional cybersecurity defenses. Adversarial machine learning techniques enable attackers to develop algorithms that can learn and adapt to counteract security measures, posing an ongoing challenge for cybersecurity professionals.
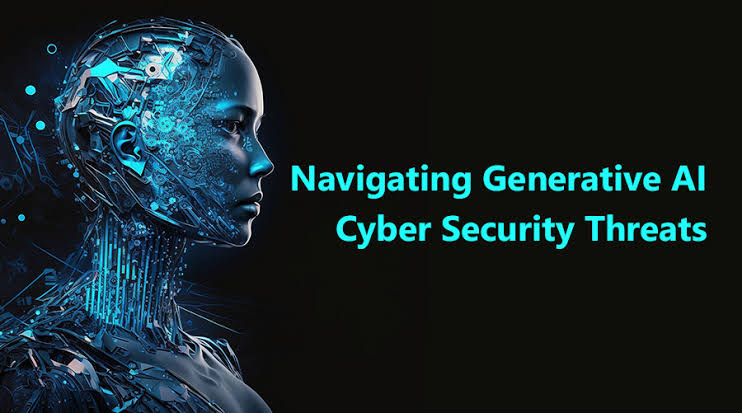
D. Spread misinformation
AI-generated fake news and social media posts can sow discord and manipulate public opinion.
E. Deepfake Technology
The use of deepfake technology, powered by generative AI, poses a substantial threat in the cybersecurity realm. Cybercriminals can create realistic audio and video deepfakes to impersonate trusted individuals, potentially tricking employees or systems into unauthorized actions, such as transferring funds or disclosing confidential information.
F. Automation of Cyber Attacks
Generative AI enables the automation of cyberattacks on a large scale. AI-driven software can rapidly exploit vulnerabilities across numerous systems before defenses can be updated, significantly increasing the efficiency and effectiveness of cyberattacks. Automated attacks can also adapt in real-time, circumventing traditional cybersecurity measures designed to thwart known attack vectors.
iv. Balancing the Scales: Mitigation Strategies
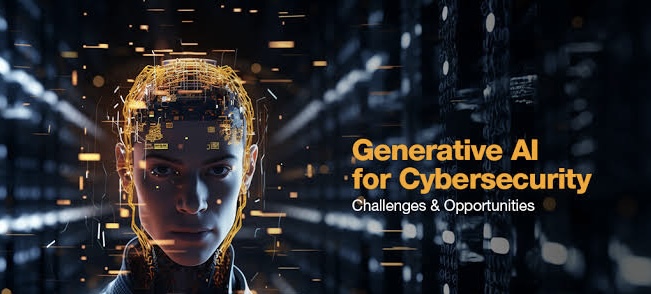
Given the dual-edged impact of generative AI on cybersecurity, it is crucial to develop comprehensive strategies to maximize its defensive benefits while mitigating its potential misuse.
Key approaches include:
o Developing Ethical AI Frameworks: As generative AI becomes integral to the cybersecurity landscape, ethical considerations come to the forefront. Striking a balance between utilizing AI for defense and managing the potential risks it poses is crucial. Ethical guidelines and regulations should be established to govern the responsible use of generative AI in both offensive and defensive cybersecurity strategies.
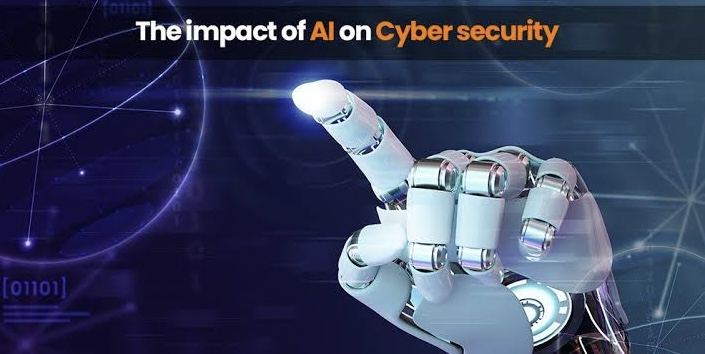
o Enhancing AI Security Measures: Investing in security technologies that can detect and neutralize AI-generated threats, including counter-AI solutions.
o International Cooperation: Collaborating globally to establish norms and regulations that govern the use of generative AI, aiming to prevent its exploitation by cybercriminals.
v. Conclusion
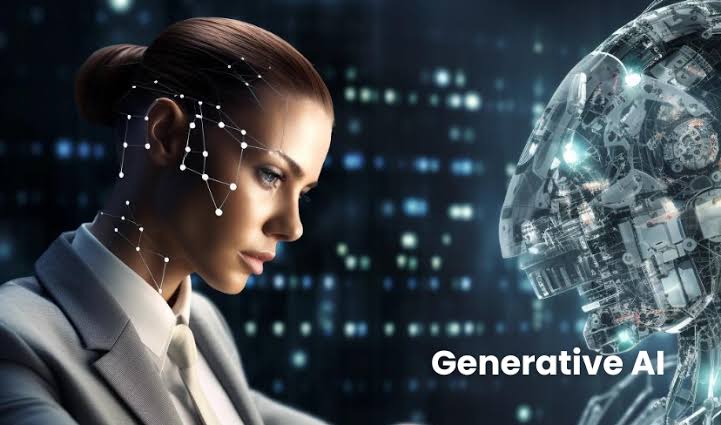
Generative AI holds transformative potential for cybersecurity, offering the means to significantly strengthen defenses while also posing the risk of enhancing cyber threats.
The challenge lies in leveraging this technology ethically and effectively, ensuring that its immense capabilities serve to protect and secure digital resources in an ever-evolving cyber threat landscape.
Navigating this terrain requires a concerted effort from policymakers, cybersecurity professionals, and AI developers to foster innovation while safeguarding against the malicious use of AI technologies.
vi. Further references
IBMhttps://www.ibm.com › en-us › reportThe CEO’s guide to generative AI: Cybersecurity
Sangforhttps://www.sangfor.com › blogGenerative AI in Cybersecurity: Offensive and …
Transputechttps://www.transputec.com › blogsGenerative AI: Revolutionising Cybersecurity