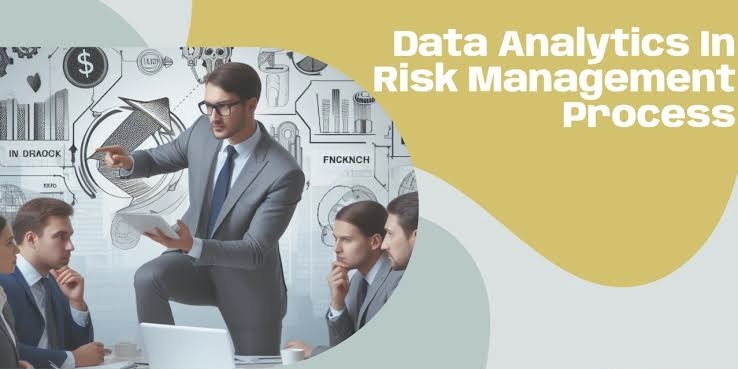
Utilizing Data Science in Risk Management: A Comprehensive Guide
In the ever-evolving landscape of business and finance, effective risk management is crucial for organizations to thrive. With the advent of technology, particularly data science, risk management has taken on a new dimension.
i. Here are some key ways data science can be used in risk management:
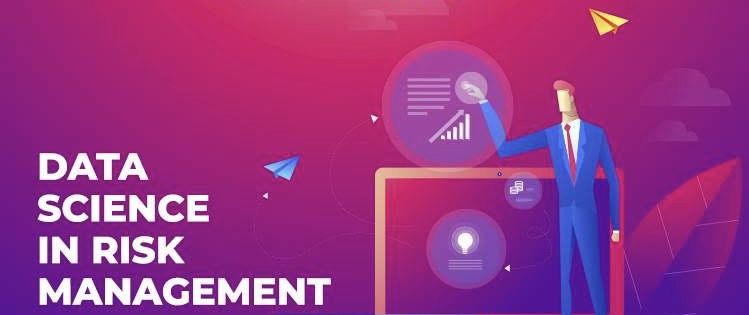
A. Enhanced Risk Identification:
o Data Mining: Analyze large datasets to uncover hidden patterns and correlations that might indicate potential risks. This could involve analyzing historical data on incidents, financial transactions, or customer behavior.
o Machine Learning: Train algorithms to identify anomalies and deviations from normal patterns that could signal emerging risks. This could involve anomaly detection in network traffic, financial transactions, or social media sentiment.
o Text Analysis: Analyze unstructured data like news articles, social media posts, and regulatory documents to identify potential threats and emerging trends that could impact your organization.
B. Improved Risk Assessment:
o Predictive Modeling: Develop models to predict the likelihood and severity of potential risks. This could involve using historical data and machine learning algorithms to predict financial losses, operational disruptions, or cyberattacks.
o Scenario Planning: Utilize data-driven simulations to assess the potential impact of different risk scenarios on your organization, allowing for better preparedness and response planning.
o Quantitative Risk Analysis: Use data to quantify the potential financial impact of different risks, enabling more informed decision-making about risk mitigation strategies.
C. Streamlined Risk Mitigation:
o Risk Prioritization: Data analysis can help prioritize risks based on their likelihood, severity, and potential impact, allowing you to focus resources on the most critical risks.
o Targeted Interventions: Develop data-driven interventions to mitigate specific risks, such as implementing targeted security controls or fraud prevention measures.
o Real-time Monitoring: Use data to monitor risk indicators in real-time and trigger automated responses to emerging threats.
D. Continuous Improvement:
o Performance Measurement: Track the effectiveness of your risk management strategies using data and metrics, allowing for continuous improvement and adaptation.
o Benchmarking: Compare your risk management performance to industry benchmarks to identify areas for improvement.
o Feedback Loops: Integrate data and feedback from various sources to continuously refine your risk models and strategies.
ii. Examples of Data Science Applications in Risk Management:
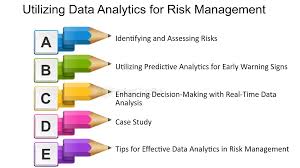
o Financial institutions: Predicting loan defaults, detecting fraudulent transactions, and managing credit risk.
o Cybersecurity: Identifying and mitigating cyberattacks, protecting sensitive data, and ensuring network security.
o Healthcare: Predicting patient readmissions, identifying potential healthcare fraud, and managing patient safety risks.
o Manufacturing: Predicting equipment failures, optimizing supply chains, and minimizing operational disruptions.
iii. Benefits of Using Data Science in Risk Management:
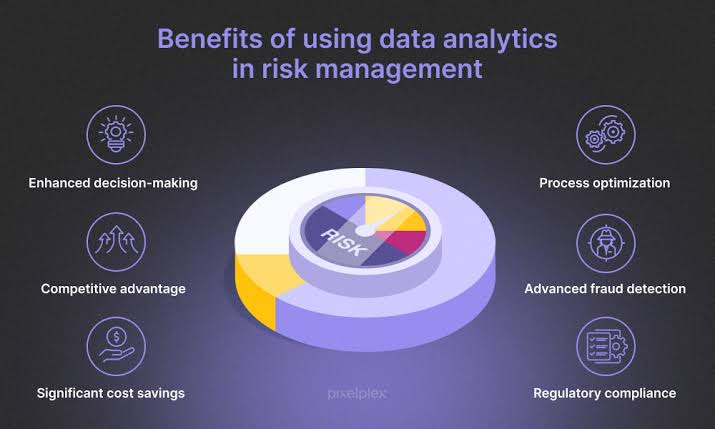
o Proactive Risk Identification: Identify and address risks before they become major problems.
o Data-Driven Decision Making: Make informed decisions about risk mitigation based on objective data.
o Improved Efficiency and Effectiveness: Allocate resources more effectively and achieve better risk management outcomes.
o Competitive Advantage: Gain a competitive advantage by proactively managing and mitigating risks.
iv. Challenges of Using Data Science in Risk Management:
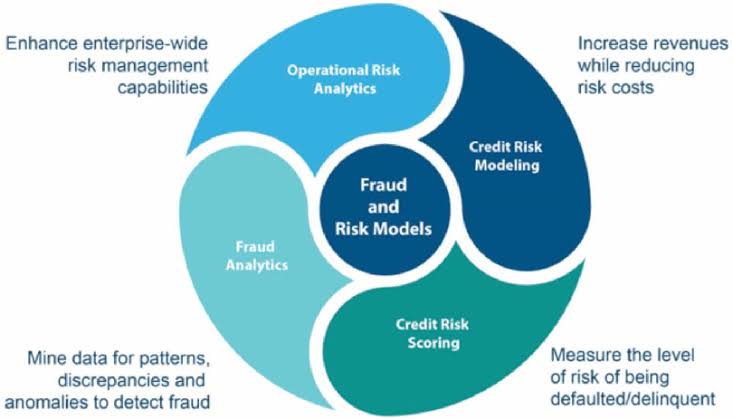
o Data Quality and Availability: Ensuring access to high-quality and relevant data is crucial for effective analysis.
o Model Development and Maintenance: Building and maintaining accurate and reliable risk prediction models requires expertise and resources.
o Organizational Integration: Integrating data science into existing risk management processes can be challenging.
v. Here’s how you can use data science in the field of risk management:
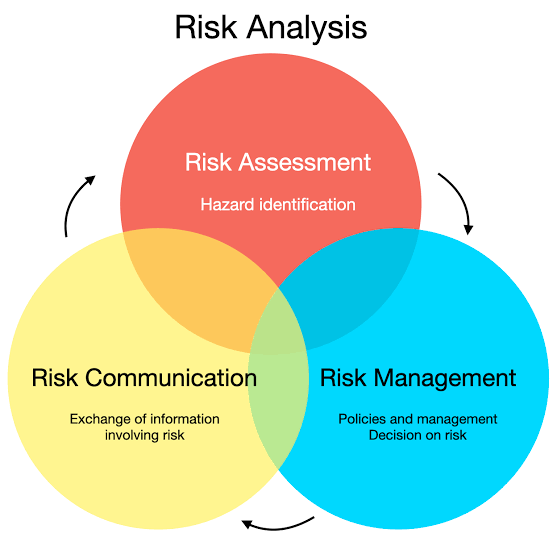
A. Understand the Problem: The first step involves a clear understanding of the risk issues that the business is facing. This could be anything from credit risk, operational risk, market risk, to liquidity risk among others.
B. Data Collection: Gather data from a variety of sources such as financial reports, transaction records, customer data, and external data sources like market feeds, social media, and news.
C. Data Cleaning and Preparation: Cleanse the data to ensure accuracy by handling missing values, addressing outliers, and correcting inconsistencies. This often involves normalizing and transforming data to be suitable for analysis.
D. Exploratory Data Analysis (EDA): Perform EDA to understand the data’s underlying patterns, correlations, and distributions. This could reveal potential risks that might not be apparent at first glance.
E. Statistical Analysis and Modeling:
o Regression Analysis: Use regression models to predict future events based on historical data.
o Time Series Analysis: For risks that have a temporal component, such as financial market risks, use time series analysis to forecast future trends.
o Survival Analysis: For analyzing the expected duration until one or more events happen, such as credit defaults or machinery failures.
F. Machine Learning:
o Classification Algorithms: To categorize risks into different buckets. For example, identifying loan applications as low, medium, or high risk.
o Clustering Algorithms: To find groups of similar risks or events without predefined labels. This can help in identifying new types of emerging risks.
o Anomaly Detection: To spot unusual patterns that may indicate fraudulent activity or operational risks.
G. Risk Quantification: Use probabilistic models to quantify risks. Techniques such as Monte Carlo simulations can estimate the impact of risk on business objectives.
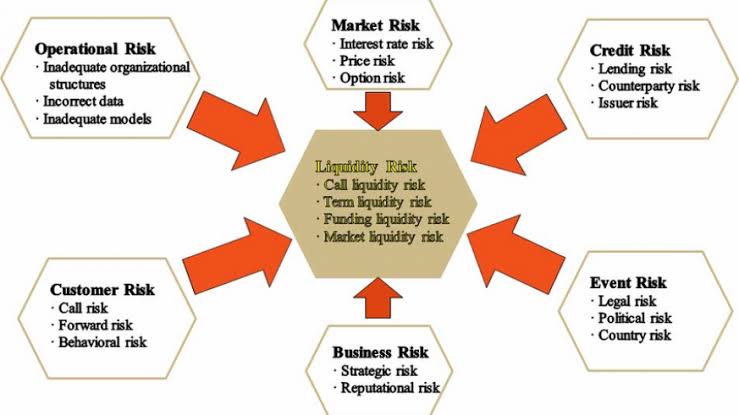
H. Risk Prediction: Deploy predictive models that can forecast potential losses or the likelihood of adverse events. This can inform better strategies for risk mitigation.
I. Prescriptive Analytics: Go beyond predictions and use optimization and simulation algorithms to recommend actions for risk mitigation.
J. Monitoring and Reporting: Develop dashboards and reports that continuously track key risk indicators. Machine learning models can be retrained with new data to ensure they remain accurate.
K. Scenario Analysis and Stress Testing: Use data science to simulate different adverse scenarios and stress-test the organization’s risk exposure.
L. Fraud Detection: Data science plays a crucial role in identifying and preventing fraudulent activities. Through advanced analytics and anomaly detection algorithms, organizations can pinpoint unusual patterns in transactions or behavior that may indicate fraudulent activities. This proactive approach is essential in mitigating financial risks associated with fraud.
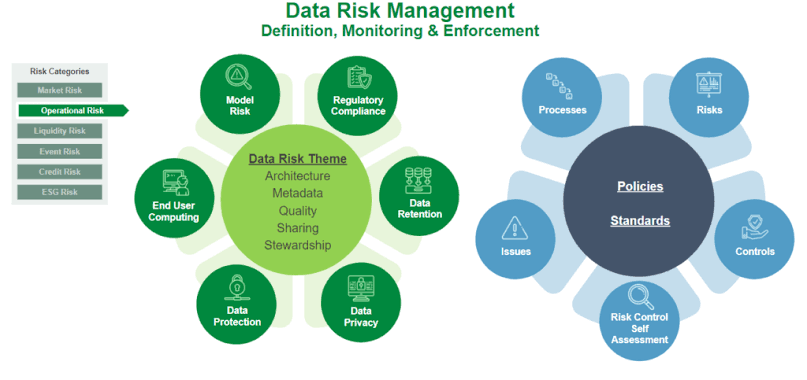
M. Decision Support Systems: Data science contributes to the development of decision support systems that empower management to make informed choices. These systems leverage real-time data analysis and provide executives with actionable insights. In risk management, this ensures timely and well-informed decisions to address emerging risks.
N. Portfolio Optimization: For organizations with diverse portfolios, data science aids in optimizing asset allocation. By analyzing the risk and return profiles of different assets, data-driven models can suggest optimal portfolio compositions to balance risk and reward. This approach helps organizations maximize returns while managing risk effectively.
O. Compliance Monitoring: Use text analytics and Natural Language Processing (NLP) for monitoring compliance with regulatory requirements by analyzing relevant documents and communications.
P. Technology Integration: Have a compatible IT infrastructure that can handle big data analytics, real-time processing, and integration with existing risk management systems.
vi. Conclusion:
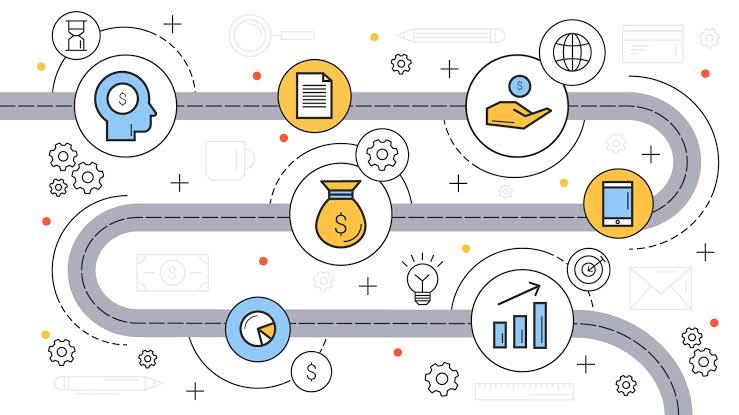
Data science provides a set of tools for actionable insights into risk management by turning raw data into an understanding that can be used to make informed decisions. The use of advanced analytics can aid in proactive risk management and help businesses avoid or mitigate potential losses.
In conclusion, the integration of data science in risk management offers a paradigm shift in how organizations identify, assess, and mitigate risks. By harnessing the power of predictive analytics, scenario analysis, and decision support systems, businesses can enhance their ability to navigate uncertainties and make strategic decisions with confidence.
Embracing data science in risk management is not just a technological advancement but a strategic imperative for modern enterprises.
vii. Further references
How Data Science Used In Risk Management? – LinkedIn
Finance Trainhttps://financetrain.com › role-of-da…Role of Data Science in Risk Management
ITChronicleshttps://itchronicles.com › BlogData Science for Risk Management
PECB Insightshttps://insights.pecb.com › how-use…How to Use Data Science in Risk Management
PW Skillshttps://pwskills.com › blog › data-sc…Role Of Data Science In Risk Management
EYhttps://www.ey.com › en_in › role-…Role of data analytics in risk management